Our Favorite Questions
Asking very simple questions often leads to discussions that give much more insight than more complex, technical questions.
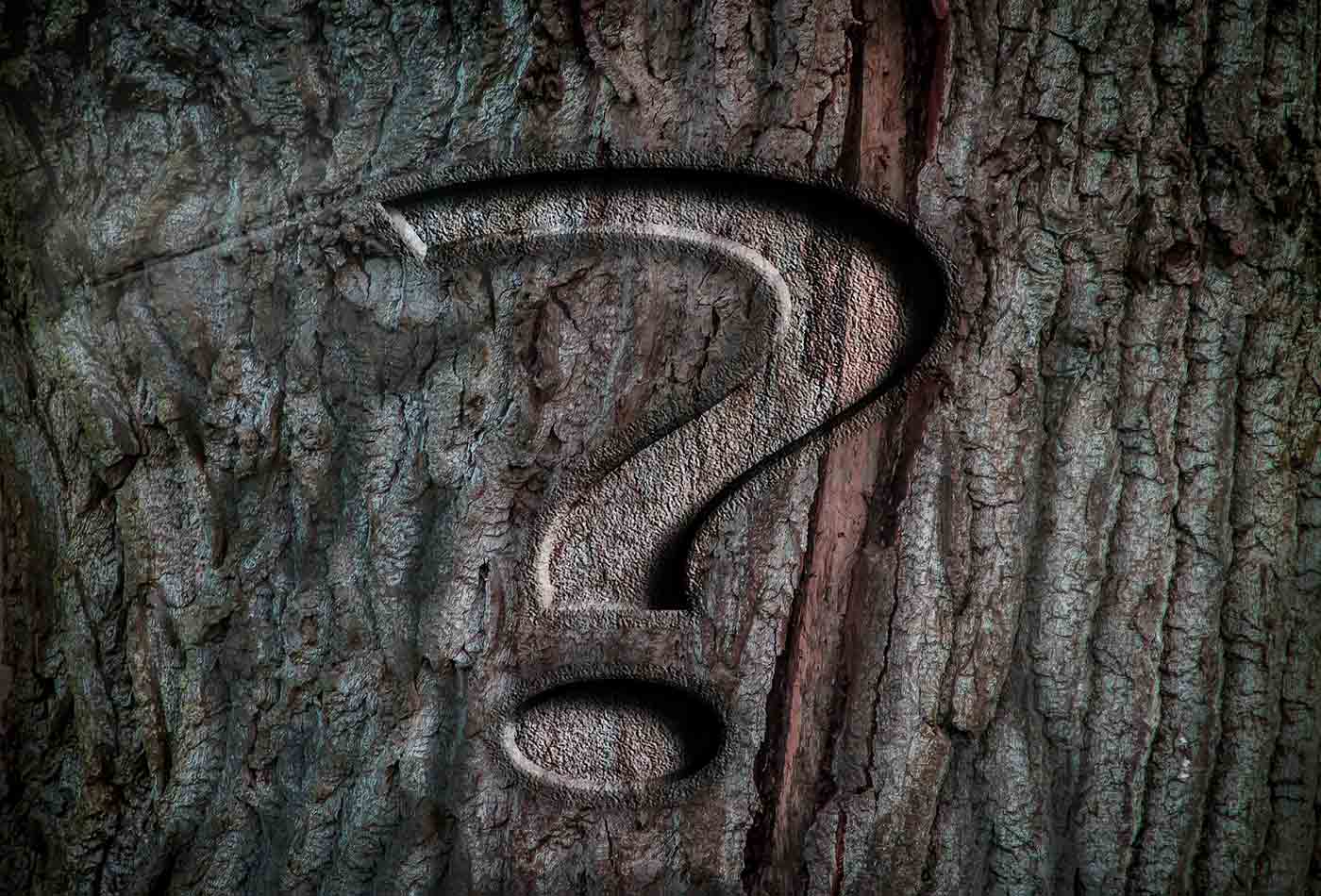
“On peut interroger n’importe qui, dans n’importe quel état; ce sont rarement les réponses qui apportent la vérité, mais l’enchaînement des questions.“
“You can interrogate anyone, no matter what their state of being. It’s rarely their answers that unveil the truth, but the sequence of questions that you have to ask.“
– Inspector Pastor in La Fée Carabine, by Daniel Pennac
The authors’ jobs all involve asking questions. A lot of questions. We do so out of genuine curiosity as well as professional necessity: Q is an ML/AI consultant, Chris is a product manager in the AI space, and Shane is an attorney. While we approach our questions from different angles because of our different roles, we all have the same goal in mind: we want to elicit truth and get people working with us to dig deeper into an issue. Preferably before things get out of hand, but sometimes precisely because they have.
A recent discussion led us down the path of our favorite questions: what they are, why they’re useful, and when they don’t work so well. We then each chose our top three questions, which we’ve detailed in this article.
We hope you’re able to borrow questions you haven’t used before, and even cook up new questions that are more closely related to your personal and professional interests.
What makes a good question?
Before we get too far, let’s explore what we mean by a “good question.”
For one, it’s broad and open-ended. It’s a lot less “did this happen?” and more “what happened?” It encourages people to share their thoughts and go deep.
There’s an implied “tell me more” in an open-ended question. Follow it with silence, and (as any professional interrogator will tell you) people will fill in extra details. They will get to what happened, along with when and how and why. They will tell a full story, which may then lead to more questions, which branch into other stories. All of this fills in more pieces to the puzzle. Sometimes, it sheds light on parts of the puzzle you didn’t know existed.
By comparison, yes/no questions implicitly demand nothing more than what was expressly asked. That makes them too easy to dodge.
Two, a good question challenges the person asking it as much as (if not more than) the person who is expected to answer. Anyone can toss out questions at random, in an attempt to fill the silence. To pose useful questions requires that you first understand the present situation, know where you want to wind up, and map out stepping-stones between the two.
Case in point: the Daniel Pennac line that opened this piece was uttered by a detective who was “interviewing” a person in a coma. As he inspected their wounds, he asked more questions to explore their backstory, and that helped him to piece together his next steps of the investigation. Perhaps Inspector Pennac was inspired by Georg Cantor, who once said: “To ask the right question is harder than to answer it.”
Three, a good question doesn’t always have a right answer. Some of them don’t have any answer at all. And that’s fine. Sometimes the goal of asking a question is to break the ice on a topic, opening a discussion that paints a larger picture.
Four, sometimes a question is effective precisely because it comes from an unexpected place or person. While writing this piece, one author pointed out (spoiler alert) that the attorney asked all of the technical questions, which seems odd, until you realize that he’s had to ask those because other people did not. When questions seem to come out of nowhere—but they are really born of experience—they can shake people out of the fog of status quo and open their eyes to new thoughts.
A brief disclaimer
The opinions presented here are personal, do not reflect the view of our employers, and are not professional product, consulting, or legal advice.
The questions
What does this company really do?
Source: Q
The backstory: This is the kind of question you sometimes have to ask three times. The first time, someone will try to hand you the company’s mission statement or slogan. The second time, they’ll provide a description of the company: industry vertical, size, and revenue. So you ask again, this time with an emphasis on the really. And then you wait for the question to sink in, and for the person to work backwards from all of the company’s disparate activities to see what it’s all truly for. Which will be somewhere between the raison d’etre and the sine qua non.
Taking the time to work this out is like building a mathematical model: if you understand what a company truly does, you don’t just get a better understanding of the present, but you can also predict the future. It guides decisions such as what projects to implement, what competitors to buy, and whom to hire into certain roles.
As a concrete example, take Amazon. Everyone thinks it’s a store. It has a store, but at its core, Amazon is a delivery/logistics powerhouse. Everything they do has to end with your purchases winding up in your hot little hands. Nothing else they do matters—not the slick website, not the voice-activated ordering, not the recommendation engine—unless they get delivery and logistics down.
How I use it: I explore this early in a consulting relationship. Sometimes even early in the sales cycle. And I don’t try to hide it; I’ll ask it, flat-out, and wait for people to fill the silence.
Why it’s useful: My work focuses on helping companies to start, restart, and assess their ML/AI efforts. Understanding the company’s true purpose unlocks the business model and sheds light on what is useful to do with the data. As a bonus, it can also highlight cases of conflict. Because sometimes key figures have very different ideas of what the company is and what it should do next.
When it doesn’t work so well: This question can catch people off-guard. Since I work in the AI space, people sometimes have a preconceived notion that I’ll only talk about data and models. Hearing this question from an ostensibly technical person can be jarring… though, sometimes, that can actually help the conversation along. So it’s definitely a double-edged sword.
What is a bad idea?
Source: Chris
The backstory: Ideation is about coming up with the “best” ideas. What is the best way to solve this problem? What is the most important? What is best for the business?
The problem with “best” is that it is tied up with all of the biases and assumptions someone already has. To get to what really matters we have to understand the edge of what is good or bad. The gray area between those tells you the shape of the problem.
Half the time this question will give you real, bad ideas.
What has been surprising to me is that the other half of the time, the so-called “bad” idea is really a “good” idea in disguise. You just have to relax certain assumptions. Often these assumptions were just set at some point without a reason or much to back it up.
How I use it: I like to ask this after going through a lot of the “best” questions in an ideation session. It can be adapted to focus on different types of “bad,” like “stupid,” “wasteful,” and “unethical.” Ask follow up questions about why they believe the idea is “bad” and why it might actually be “good.”
Why it’s useful: How can you truly know what is good without also knowing what is bad?
When it doesn’t work so well: When I was a design consultant working for clients in highly regulated industries (.e.g banking, insurance, etc.), I found this can be a difficult question to ask. In those cases you will need to get your legal team to either grant the attorney/client privilege to ask the questions, or ask the prompt/response in such a way that it protects people in the conversation.
How did you obtain your training data?
Source: Shane
The backstory: In the early days of ML training data, companies and research teams frequently used “some stuff we found on the Internet” as a source for training data. This approach has two problems: (1) there may not be an appropriate license attached to the data, and (2) the data may not be a good representative sample for the intended use. It’s worth noting that the first issue is not just limited to images collected from the Internet. In recent years a number of research datasets (including Stanford’s Brainwash, Microsoft’s MS Celeb, and Duke’s MTMC) were withdrawn for reasons including a lack of clarity around the permission and rights granted by people appearing in the datasets. More recently, at least one company has earned itself significant PR and legal controversy for collecting training data sources from social media platforms under circumstances that were at least arguably a violation of both the platform’s terms of service and platform users’ legal rights.
The safest course of action is also the slowest and most expensive: obtain your training data as part of a collection strategy that includes efforts to obtain the correct representative sample under an explicit license for use as training data. The next best approach is to use existing data collected under broad licensing rights that include use as training data even if that use was not the explicit purpose of the collection.
How I use it: I like to ask this as early as possible. You don’t want to invest your time, effort, and money building models only to later realize that you can’t use them, or that using them will be much more expensive than anticipated because of unexpected licenses or royalty payments. It’s also a good indirect measure of training data quality: a team that does not know where their data originated is likely to not know other important details about the data as well.
Why it’s useful: No matter how the data is collected, a review by legal counsel before starting a project—and allow me to emphasise the word before—can prevent significant downstream headaches.
When it doesn’t work so well: This question is most useful when asked before the model goes into production. It loses value once the model is on sale or in service, particularly if it is embedded in a hardware device that can’t be easily updated.
What is the intended use of the model? How many people will use it? And what happens when it fails?
Source: Shane
The backstory: One of the most interesting aspects of machine learning (ML) is its very broad applicability across a variety of industries and use cases. ML can be used to identify cats in photos as well as to guide autonomous vehicles. Understandably, the potential harm caused by showing a customer a dog when they expected to see a cat is significantly different from the potential harm caused by an autonomous driving model failing to properly recognize a stop sign. Determining the risk profile of a given model requires a case-by-case evaluation but it can be useful to think of the failure risk in three broad categories:
- “If this model fails, someone might die or have their sensitive data exposed” — Examples of these kinds of uses include automated driving/flying systems and biometric access features. ML models directly involved in critical safety systems are generally easy to identify as areas of concern. That said, the risks involved require a very careful evaluation of the processes used to generate, test, and deploy those models, particularly in cases where there are significant public risks involved in any of the aforementioned steps.
- “If this model fails, someone might lose access to an important service” — Say, payment fraud detection and social media content detection algorithms. Most of us have had the experience of temporarily losing access to a credit card for buying something that “didn’t fit our spending profile.” Recently, a law professor who studies automated content moderation was suspended … by a social media platform’s automated content moderation system. All this because they quoted a reporter who writes about automated content moderation. These kinds of service-access ML models are increasingly used to make decisions about what we can spend, what we can say, and even where and how we can travel. The end-user risks are not as critical as in a safety or data protection system, but their failure can represent a significant reputation risk to the business that uses them when the failure mode is to effectively ban users from a product or service. It is important for companies employing ML in these situations to understand how this all fits into the overall risk profile of the company. They’d do well to carefully weigh the relative merit of using ML to augment existing controls and human decision-making versus replace those controls and leave the model as the sole decision-maker.
- “If this model fails, people may be mildly inconvenienced or embarrassed” — Such systems include image classifiers, recommendation engines, and automated image manipulation tools. In my experience, companies significantly understate the potential downside for ML failures that, while only inconvenient to individual users, can carry significant PR risk in the aggregate. A company may think that failures in a shopping recommendation algorithm are “not a big deal” until the algorithm suggests highly inappropriate results to millions of users for an innocuous and very common query. Similarly, employees working on a face autodetection routine for a camera may think occasional failures are insignificant until the product is on sale and users discover that the feature fails to recognize faces with facial hair, or a particular hairstyle, or a particular range of skin color.
How I use it: I use this question to determine both the potential risk from an individual failure and the potential aggregate risk from a systemic failure. It also feeds back into my question about training data: some relatively minor potential harms are worth additional investment in training data and testing if they could inconvenience millions, or billions, of users or create a significant negative PR cycle for a company.
Why it’s useful: This is the sort of question that gets people thinking about the importance of their model in the overall business. It can also be a helpful guide that companies invest in such a model, and the kinds of business processes that are amenable to models. Remember that models that work nearly perfectly can still fail spectacularly in unusual situations.
When it doesn’t work so well: We don’t always have the luxury of time or accurate foresight. Sometimes a business does not know how a model will be used: a model is developed for Product X and repurposed for Product Y, a minor beta feature suddenly becomes an overnight success, or a business necessity unexpectedly forces a model into widespread production.
What’s the cost of doing nothing?
Source: Q
The backstory: A consultant is an agent of change. When a prospect contacts me to discuss a project, I find it helpful to compare the cost of the desired change to the cost of another-change or even to the cost of the not-change. “What happens if you don’t do this? What costs do you incur, what exposures do take on now? And six months from now?” A high cost of doing nothing means that this is an urgent matter.
Some consultants will tell you that a high cost of doing nothing is universally great (it means the prospect is ready to move) and a low cost is universally bad (the prospect isn’t really interested). I see it differently: we can use that cost of doing nothing as a guide to how we define the project’s timeline, fee structure, and approach. If the change is extremely urgent—a very high cost of doing nothing—it may warrant a quick fix now, soon followed by a more formal approach once the system is stable. A low cost of doing nothing, by comparison, means that we can define the project as “research” or “an experiment,” and move at a slower pace.
How I use it: I will ask this one, flat-out, once a consulting prospect has outlined what they want to do.
Why it’s useful: Besides helping to shape the structure of the project, understanding the cost of doing nothing can also shed light on the prospect’s motivations. That, in turn, can unlock additional information that can be relevant to the project. (For example, maybe the services I provide will help them reach the desired change, but that change won’t really help the company. Perhaps I can refer them to someone else in that case.)
When it doesn’t work so well: Sometimes people don’t have a good handle on the risks and challenges they (don’t) face. They may hastily answer that this is an urgent matter when it’s not; or they may try to convince you that everything is fine when you can clearly see that the proverbial house is on fire. When you detect that their words and the situation don’t align, you can ask them to shed light on their longer-term plans. That may help them to see the situation more clearly.
How would we know we are wrong?
Source: Chris
The backstory: This is something that was inspired from the intersection of an incredibly boring decision-science book and roadmap planning. Decision trees and roadmaps are very useful when building out the possible spaces of the future. However, for both decision trees and roadmaps we are usually overly optimistic in how we will proceed.
We fail at properly considering failure.
To appropriately plan for the future we must consider the different ways we can be wrong. Sometimes it will be at a certain decision point (“we didn’t get enough signups to move forward”) or an event trigger (“we see too many complaints”).
If we consider this wrong-ness and the possible next step, we can start to normalize this failure and make better decisions.
How I use it: It’s best to ask this when you find that certainty is at a high point for the project. More often than not, people don’t consider ways to detect that they need to change course.
Why it’s useful: You build a map into the future based on what you can detect. This helps make hard decisions easier because you are effectively practicing the decision process before you are in the heat of the moment.
When it doesn’t work so well: When things are currently going “wrong” it can be a sensitive subject for people. I’ve found it is easier to talk about how to get out of a current wrong situation than considering additional future situations.
What upstream obligations do you have, and what downstream rights do you want to retain?
Source: Shane
The backstory: Imagine you employ a vendor to provide or enrich your training data, or you pay for consulting services related to ML. What happens to the information used by the vendors to build your product? Their downstream rights there run the gamut from “absolutely nothing” to “retaining a full copy of the training data, labels, trained models, and test results.” The median position, in my observation, tends to be that the vendor retains control of any new techniques and information derived from the work that would be useful in general, such as new methods of programmatically applying error correction to a trained model, but not the specific data used to train the model or the resulting trained model.
From the customer perspective, downstream rights are tied to competition/cost tradeoffs and the rights associated with training data. A company that considers ML a competitive advantage likely will not want their models or derivative data available to competitors, and they must balance this against the business consideration that vendors which retain downstream rights typically charge lower fees (because reselling that data or models can be a source of revenue). In addition, training data usually comes with contractual limitations and customers of ML services need to ensure they are not granting downstream rights that they don’t have in their upstream agreements. Finally, some kinds of training data, such as medical records or classified government data, may forbid unauthorized access or use in systems that lack adequate safeguards and audit logs.
How I use it: This question is less relevant to companies that have an entirely in-house workflow (they generate their own training data, train their own models, and use models with their own employees and tools). It is highly relevant to companies that buy or sell ML services, use external vendors for part of their workflow, or handle sensitive data.
Why it’s useful: The notion of downstream rights is not a new question, nor is it specific to the ML world. Almost all vendor relationships involve delineating the intellectual property (IP) and tools that each party brings to the project, as well as the ownership of new IP developed during the project. Helping founders to recognize and establish those boundaries early on can save them a lot of trouble later.
When it doesn’t work so well: This is a question a company definitely wants to answer before they’ve provided data or services to a counterparty. These issues can be very difficult to resolve once data has been shared or work has begun.
What if …? Then …? and What next?
Source: Q
The backstory: A risk is a potential change that comes with consequences. To properly manage risk—to avoid those consequences—you need to identify those changes in advance (perform a risk assessment) and sort out what to do about them (devise your risk mitigation plans). That’s where this trio of questions comes in: “What if?” is the key to a risk assessment, as it opens the discussion on ways a project may deviate from its intended path. “Then?” explores the consequences of that deviation. The “What next?” starts the discussion on how to handle them.
“What if … our data vendor goes out of business? Then? Our business is hamstrung. What next? We’d better have a backup data vendor in the wings. Or better yet, keep two vendors running concurrently so that we can switch over with minimal downtime.”
“What if … something changes, and the model’s predictions are wrong most of the time? Then? We’re in serious trouble, because that model is used to automate purchases. What next? We should implement monitors around the model, so that we can note when it’s acting out of turn. We should also add a ‘big red button’ so that a person can quickly, easily, and completely shut it down if it starts to go haywire.”
How I use it: Once we’ve sorted out what the client wants to achieve, I’ll round out the picture by walking them through some “What if? Then? What next?” scenarios where things don’t work out.
Why it’s useful: It’s too easy to pretend the not-intended outcomes don’t exist if you don’t bring them up. I want my clients to understand what they’re getting into, so they can make informed decisions on whether and how to proceed. Going through even a small-scale risk assessment like this can shed light on the possible downside loss that’s lurking alongside their desired path. All of that risk can weigh heavily on their investment, and possibly even wipe out any intended benefit.
When it doesn’t work so well: The business world, especially Western business culture, has a strange relationship with positive attitudes. This energy can be infectious and it can help to motivate a team across the finish line. It can also convince people to pretend that the non-intended outcomes are too remote or otherwise not worth consideration. That’s usually when they find out, the hard way, what can really go wrong.
How to handle this varies based on your role in the company, internal company politics, your ability to bring about change, and your ability to weather a storm.
A random question
Source: Chris
The backstory: The most important question is one that isn’t expected. It is one that leads to unexpected answers. We don’t have dialog for dialog sake; we do it to learn something new. Sometimes the thing we learn is that we aren’t aligned.
I’ve found that the most unexpected thing is something that we wouldn’t choose based on our current thought process. Randomly choosing a question from a collection appropriate for your domain is really valuable. If you are building something for the web, what kinds of questions could you ask about a web project? This is helpful when the checklists of things to do get too large to try all of them. Pick a few at random.
You can take it a step further and pick questions from outside of your domain. This can simply be a list of provocations that require a high amount of interpretation by you to make sense. This is because randomness doesn’t work without the lens of human intuition.
Randomness without this intuition is just garbage. We do the work to bridge from random questions to some new idea related to our problem. We build the analogies in our mind even when something is seemingly not connected at first.
How I use it: When you find that you keep asking the same questions. I have decks of cards like Oblique Strategies for provocations, Triggers for domain-specific questions, and others that can provide randomness. Domain-specific random questions can also be very impactful. Eventually, I expect models like GPT-n to provide appropriate random questions to prompts.
Why it’s useful: Even with all of the questions we ask to get out of bias, we are still biased. We still have assumptions we don’t realize. Randomness doesn’t care about your biases and assumptions. It will ask a question that you think on the surface is stupid, but when you think about it is important.
When it doesn’t work so well: With teams that are high on certainty they may think of the random question as a toy or distraction. The people I’ve found to be incredibly confident in their world trivialize the need to question bias. They will even try to actively subvert the process sometimes. If you hide the fact that a question was randomly chosen, it can go over better.
In search of the bigger picture …
If you’re collecting facts—names, numbers, times—then narrow questions will suffice. But if you’re looking to understand the bigger picture, if you want to get a meeting out of a rut, if you want people to reflect before they speak, then open-ended questions will serve you well. Doubly so when they come from an unexpected source and at an unexpected time.
The questions we’ve documented here have helped us in our roles as an AI consultant, a product manager, and an attorney. (We also found it interesting that we use a lot of the same questions, which tells us how widely applicable they are.) We hope you’re able to put our favorite questions to use in your work. Perhaps they will even inspire you to devise and test a few of your own.
One point we hope we’ve driven home is that your goal in asking good questions isn’t to make yourself look smarter. Nor is it to get the answers you want to hear. Instead, your goal is to explore a problem space, shed light on new options, and mitigate risk. With that new, deeper understanding, you’re more prepared to work on the wicked problems that face us in the workplace and in the world at large.