The state of AI adoption
An overview of adoption, and suggestions to companies interested in AI technologies.
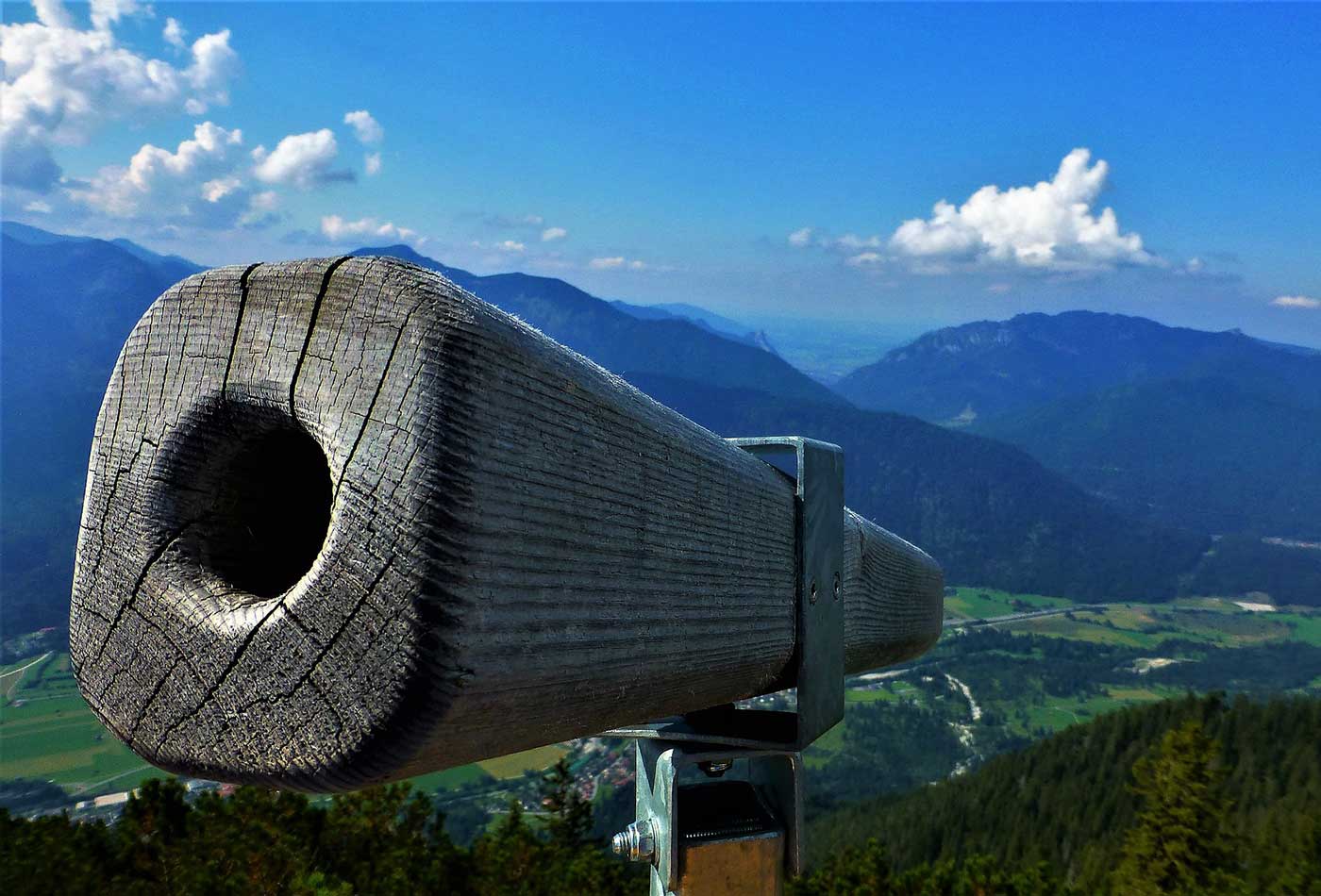
Artificial intelligence (AI) has attracted a lot of media coverage recently, and companies are rushing to figure out how AI technologies will impact them. Much of the coverage is devoted to research breakthroughs or new product offerings. But how are companies integrating AI into their underlying businesses? In this post, we share slides and notes from a talk we gave this past September at the AI Conference in San Francisco, offering an overview of the state of adoption and some suggestions to companies interested in implementing AI technologies.
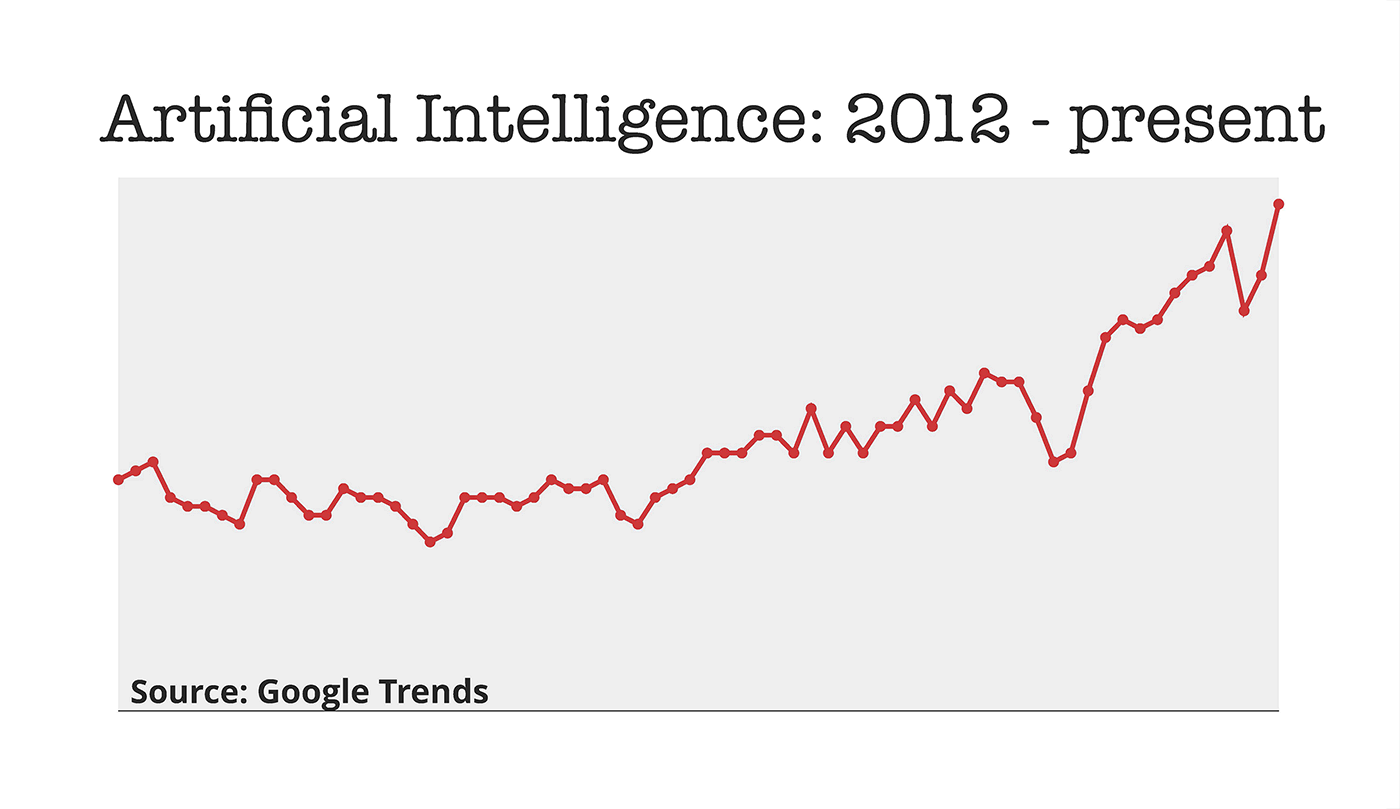
Much of the renewed interest in AI can be attributed to deep learning. Breakthroughs in deep learning (particularly as applied to computer vision and speech) have excited people about the possibilities of modern AI applications. The result is that companies are beginning to examine applications of deep learning to data they are familiar with, while considering data types (such as images, audio, video) of which they have yet to take advantage.
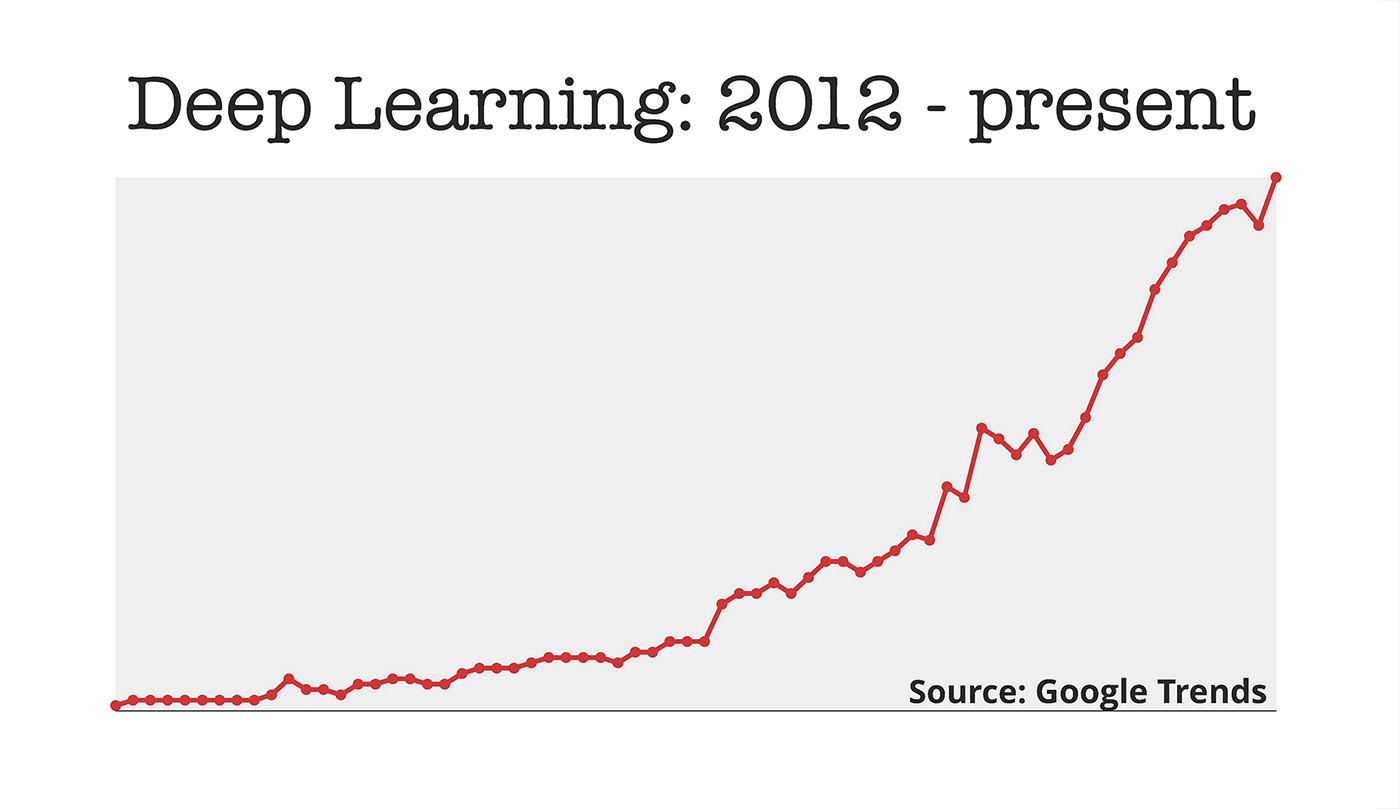
In a later part of this post, we will sketch out a typical technology stack for intelligent applications. Within the space of algorithms, it’s important to emphasize that AI applications will rely on more than just deep learning. The recent rise of other techniques like reinforcement learning and related methods that rely on sparse feedback, have had a profound impact on how AI systems are getting built. Some of the more prominent examples of AI breakthroughs like DeepMind’s AlphaGo and Atari game playing systems relied on reinforcement learning in conjunction with deep learning or other methods.
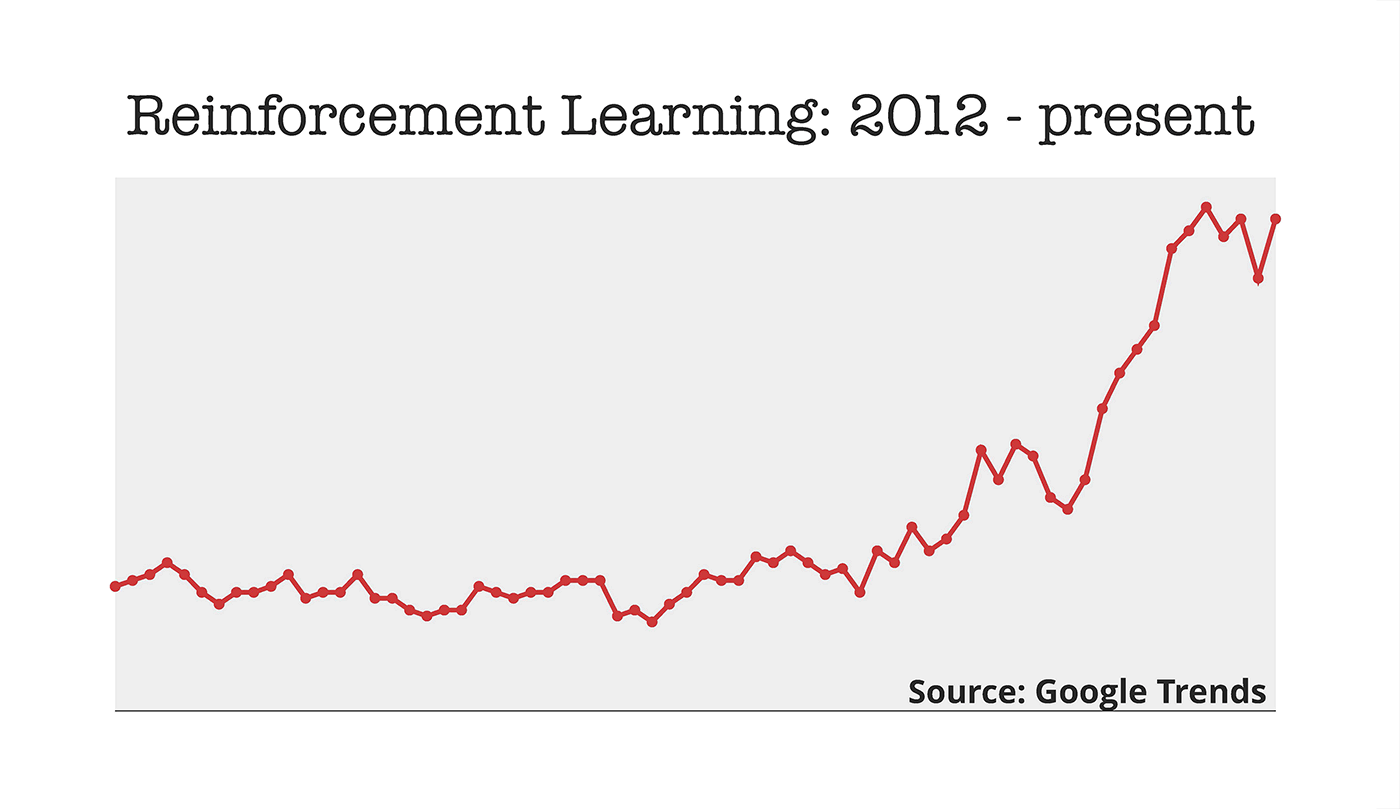
As companies take notice and begin investing in tools and methods central to AI, there is immense industry demand for AI talent, particularly around deep learning.
We are also beginning to see the impact of AI on perception of corporate value in public markets: the number of companies that want to be associated with AI is rising rapidly. A widely shared chart from early this year showed a large spike in the number of companies who mentioned “Artificial Intelligence” during their quarterly earnings call.
The fact that companies want to embrace AI technologies is not surprising. A recent OECD study found that the gap between the “most productive” and the “least productive” has widened over the last decade. This comprehensive study examined firms, in both manufacturing and services, across 24 countries over an extended period (1997-2014). So, our main message to companies is: “Don’t fall behind!” You need to begin to explore and learn how AI can potentially help your underlying businesses.
What is the level of adoption of AI technologies today? A recent survey of 3,000 executives, managers, and analysts conducted by MIT Sloan Management Review suggests that a surprising majority of companies have not yet adopted AI technologies. Less than a quarter have adopted AI technologies, while another 23% of those surveyed have some sort of pilot project in progress. In particular 54% stated that they have not started adopting AI technologies.
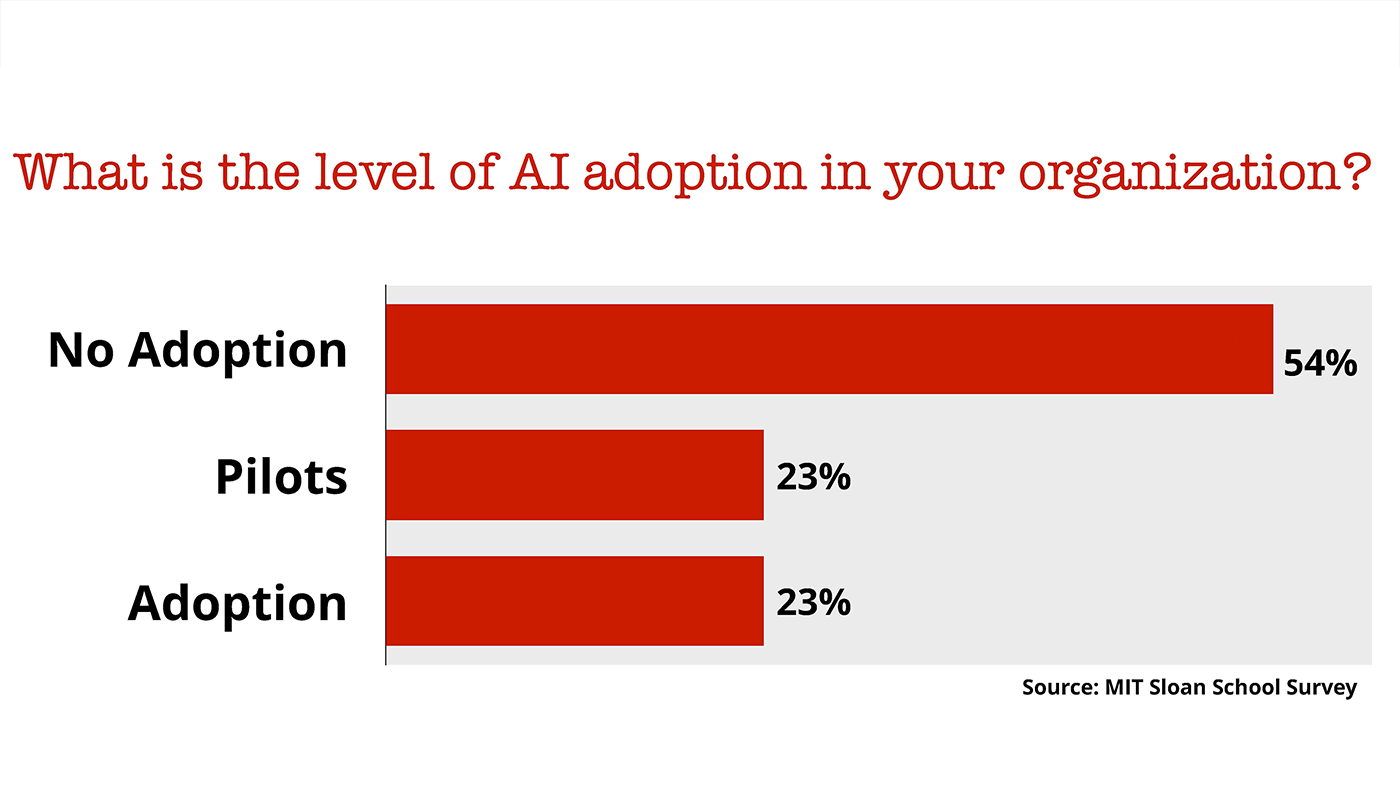
The same survey from MIT Sloan Management Review provided clues as to possible obstacles that stand in the way of increased adoption of AI.
- Organizations that understood and have already adopted AI (“Leaders”) cited a talent gap, competing investment priorities, and concerns about security as their main barriers.
- Meanwhile, companies that haven’t yet adopted AI (“Passives”) cited the need to identify business use cases, lack of management support, and limited technology capabilities as their main challenges.
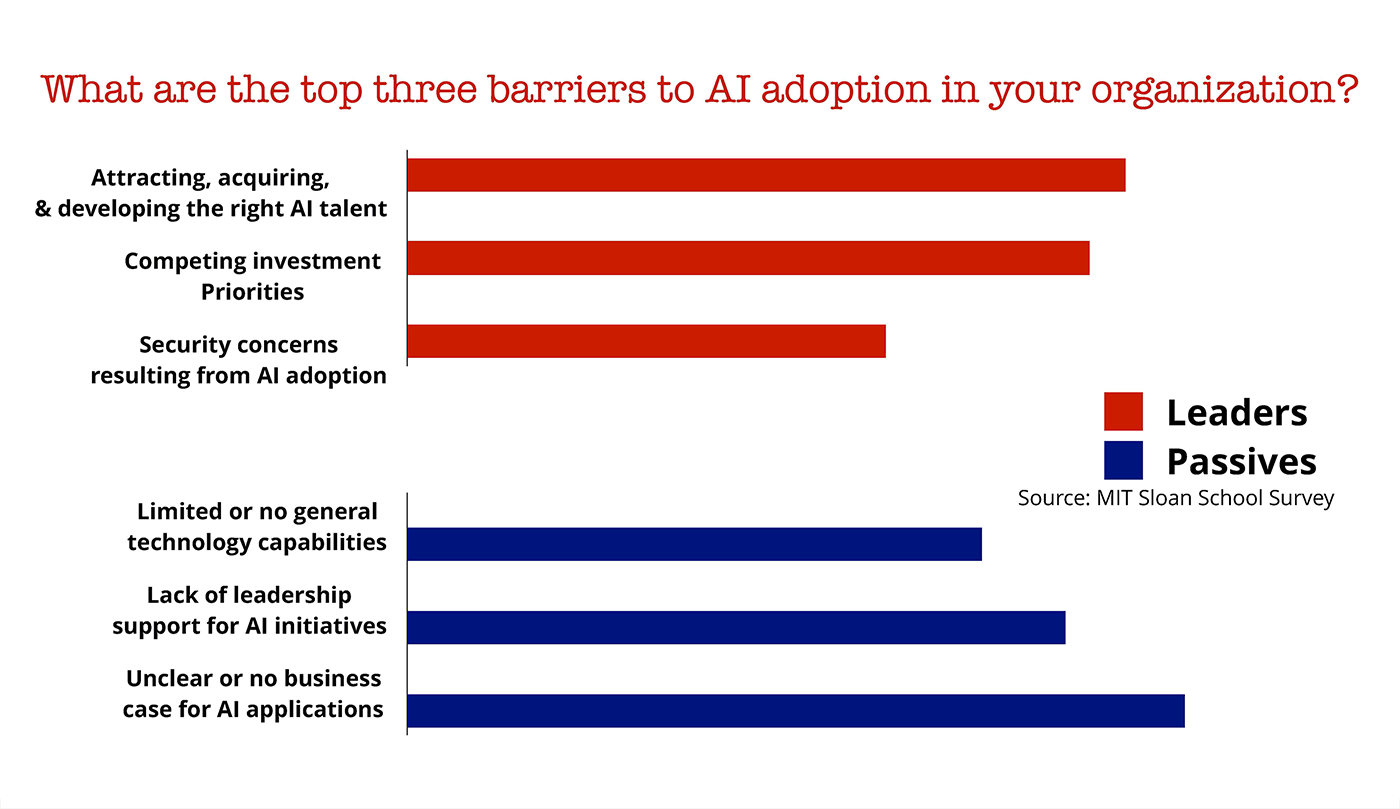
As with any new technology, AI really is still about people, particularly those who can turn AI technology into end application value. LinkedIn recently conducted a study that attempted to measure the number of users who have AI related technologies and skills in their profile. They found that, by a wide margin, the U.S. has the largest AI talent pool.
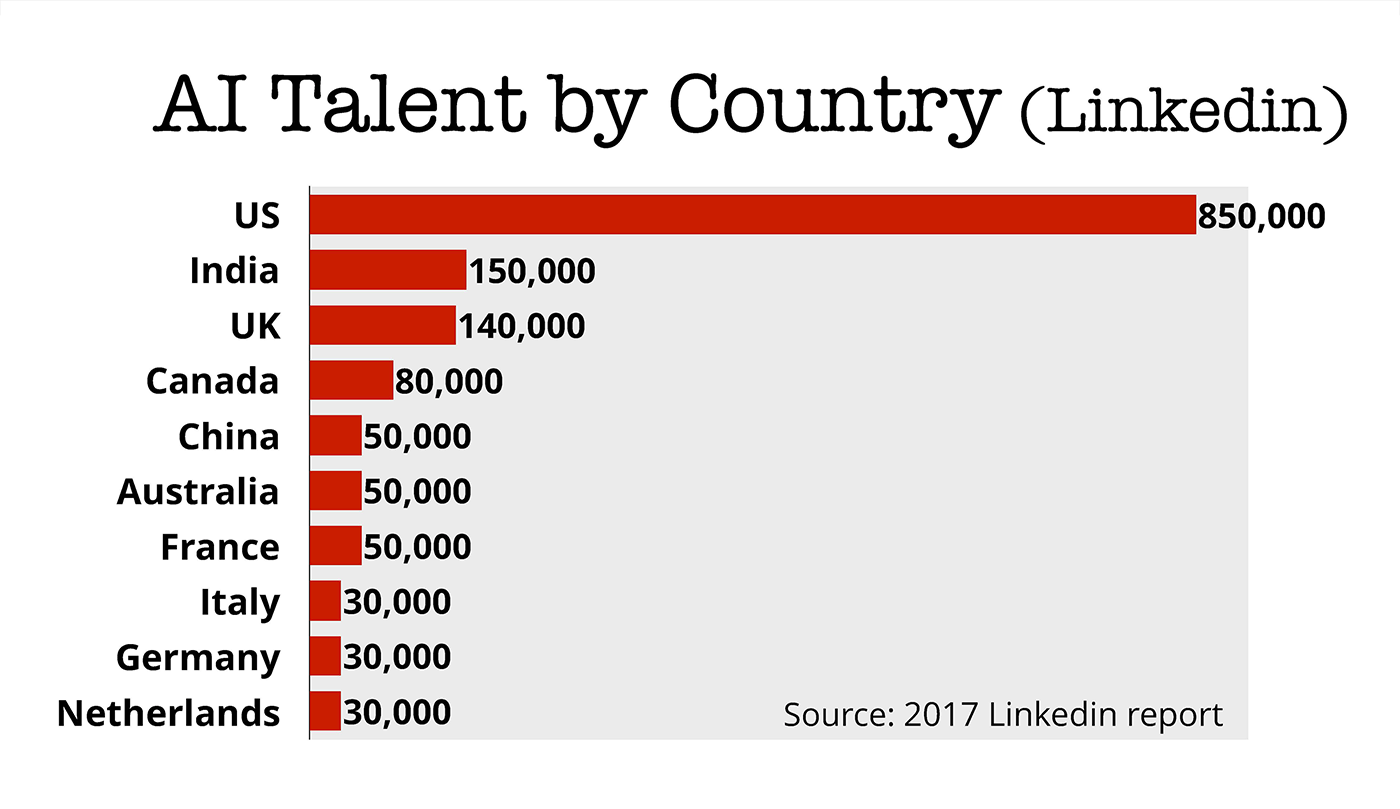
Perhaps this isn’t that surprising, given that LinkedIn has the most number of users in the U.S. Once we normalize by the number of LinkedIn users, the U.S. remains among the top countries, but other nations can boast that they have more AI talent per capita.
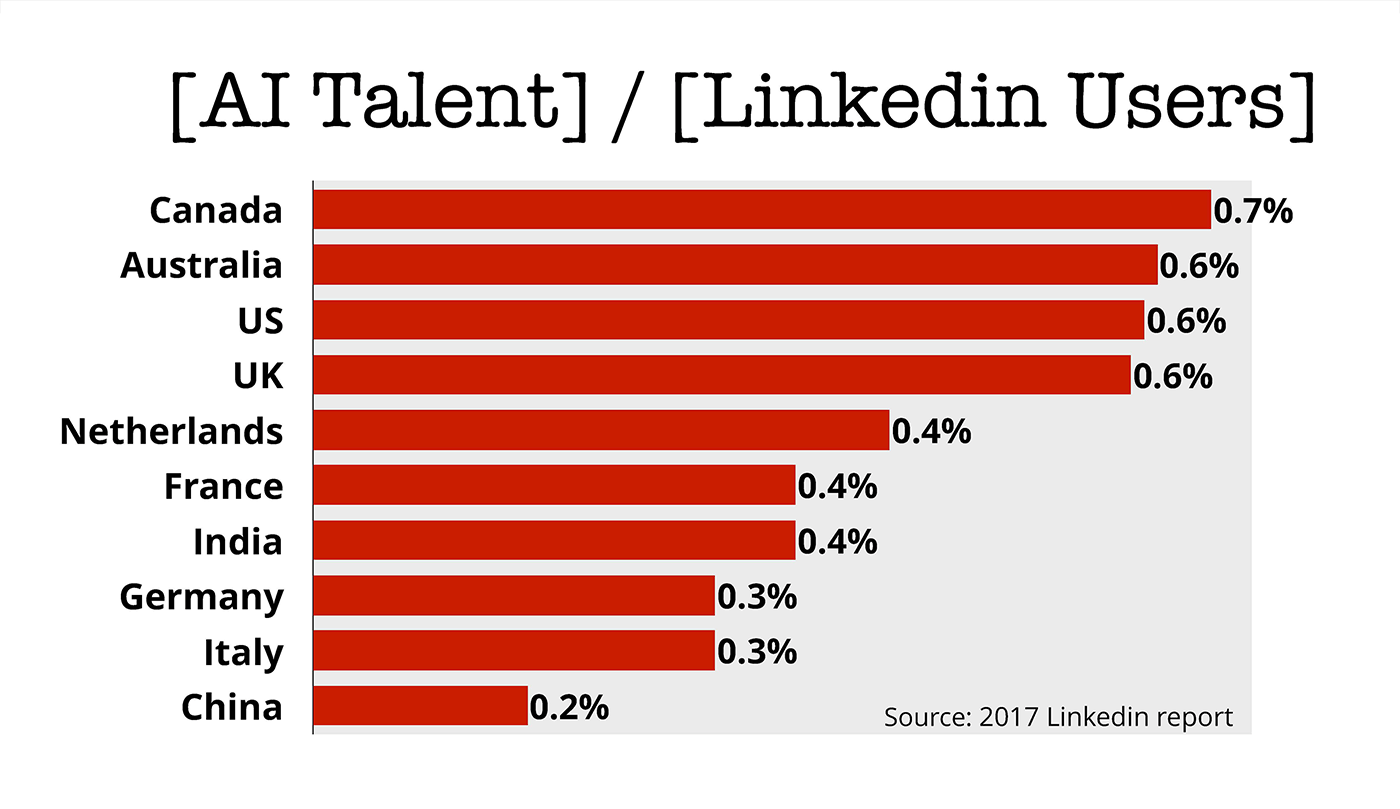
The growing interest in AI isn’t lost on job seekers. An increasing number of them are searching for positions that will allow them to use and learn AI technologies.
We ourselves have seen a surge in interest in AI-related topics among enterprises. Usage of deep learning content on our Safari Learning Platform has been growing steadily over the last three years.
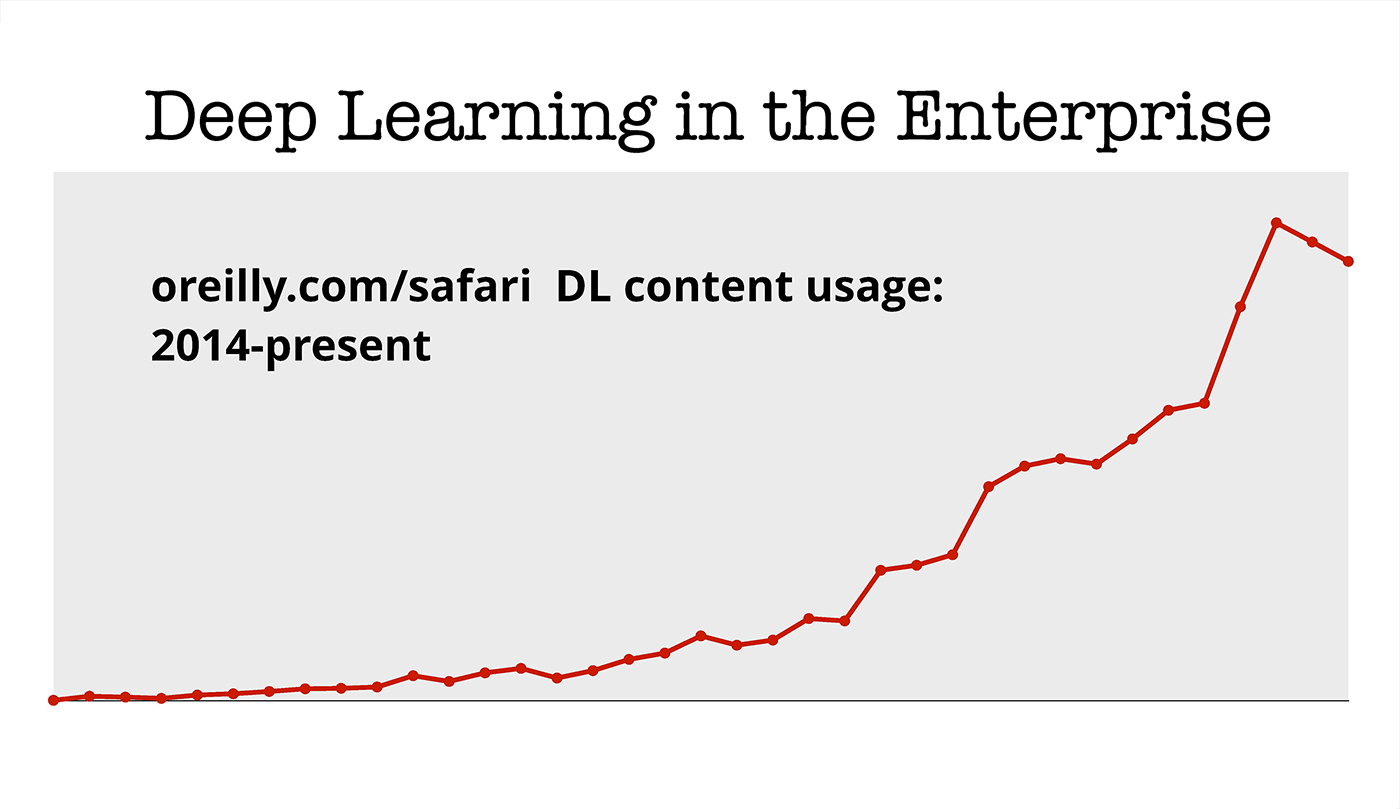
As you begin your journey, it’s useful to understand some of the key components of an AI application stack. Machine learning is an important piece, but it isn’t the only component found in intelligent applications. A recent UC Berkeley RISE Lab paper on next-generation AI applications outlines a research agenda involving systems, security, and computer architecture. A common representation of what the computing stack looks like for artificial intelligence involves an agent (interacting with, and exploring an environment), paired with a back-end platform for large-scale learning and simulations. Advances in hardware, software, networking, and design/UX will influence how these components develop.
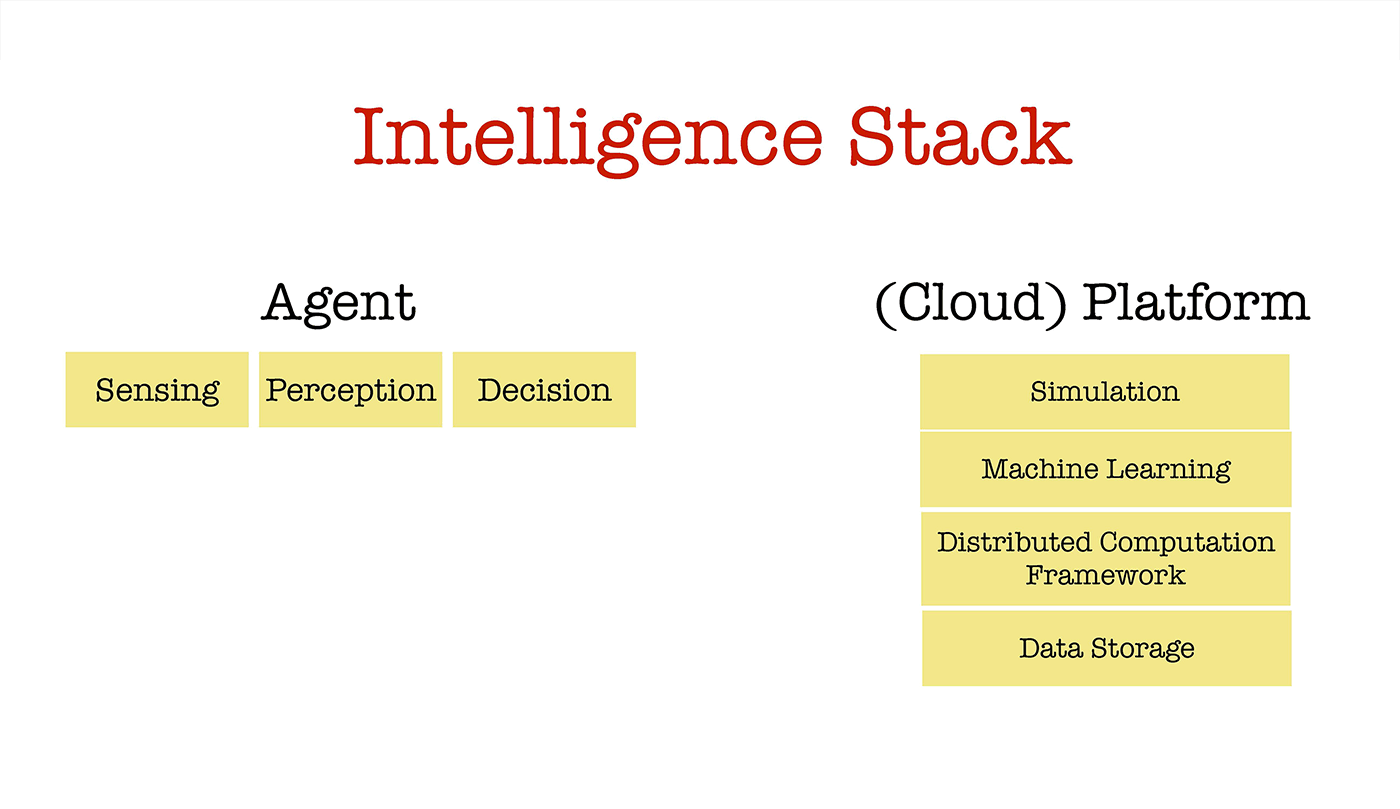
A recent article by Shaoshan Liu outlines what an intelligence stack looks like for autonomous vehicles. Machine learning is an important piece, but many other technologies and techniques come into play. Specialized (or application-specific) sensors and algorithms are critical. Just the modules for sensing and perception combine collections of hand-crafted rules, along with many recent advances in hardware and algorithms, including control theory, deep learning, computer vision, sensor fusion, and machine learning at the edge.
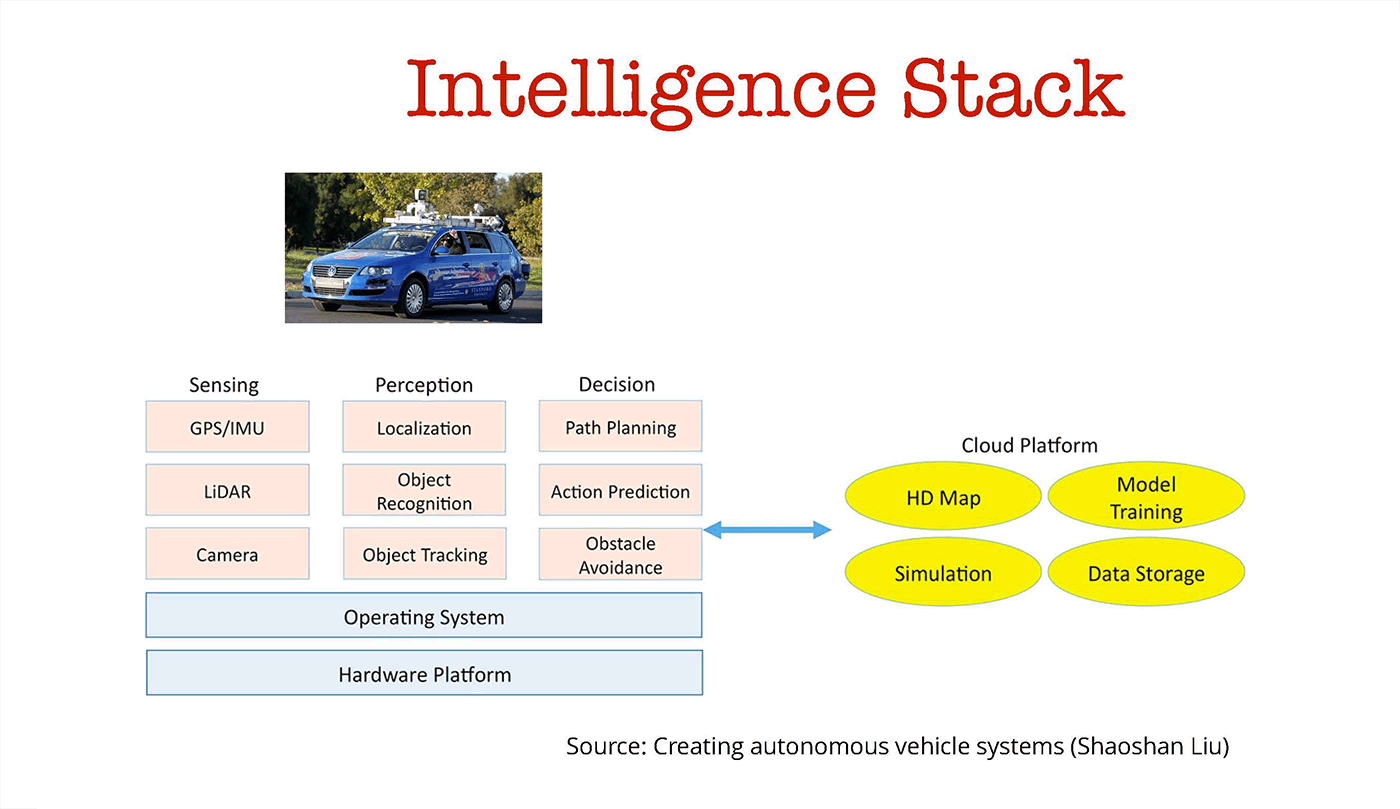
The technology stack we outlined looks challenging for a typical enterprise to build. The good news is that startups are hard at work developing solutions. The growth in AI tools and advances in computing combined with demand from companies, have resulted in increased funding for startups that can help companies adopt AI.
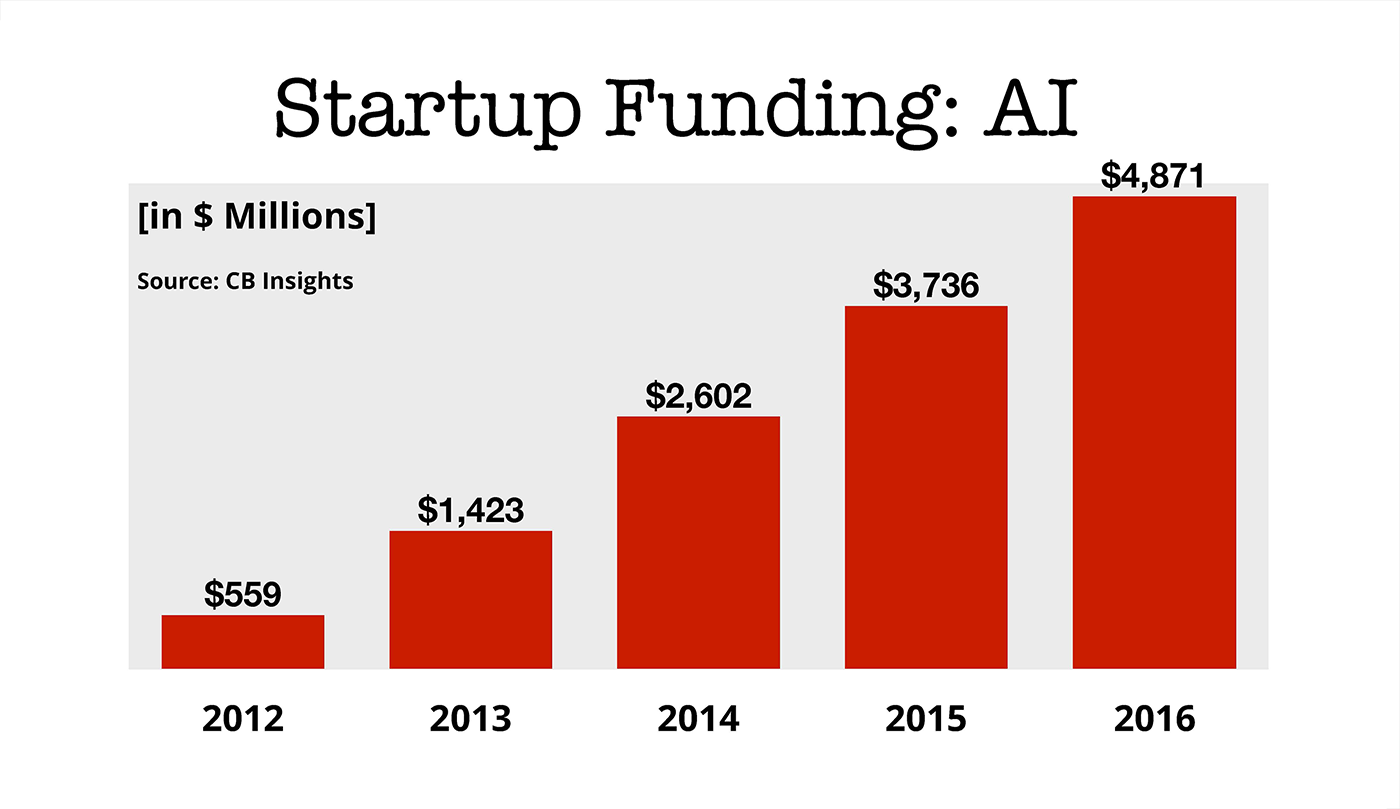
But you shouldn’t wait for startups that have the end-to-end solutions you desire. You can begin by educating yourself on the current state of tools and technologies, identifying use cases in your domain or industry, and starting with small pilot projects.