Put machine learning to work in the real world
The sessions and training courses at Strata Data San Jose 2018 will focus on practical use cases of machine learning for data scientists, engineers, managers, and executives.
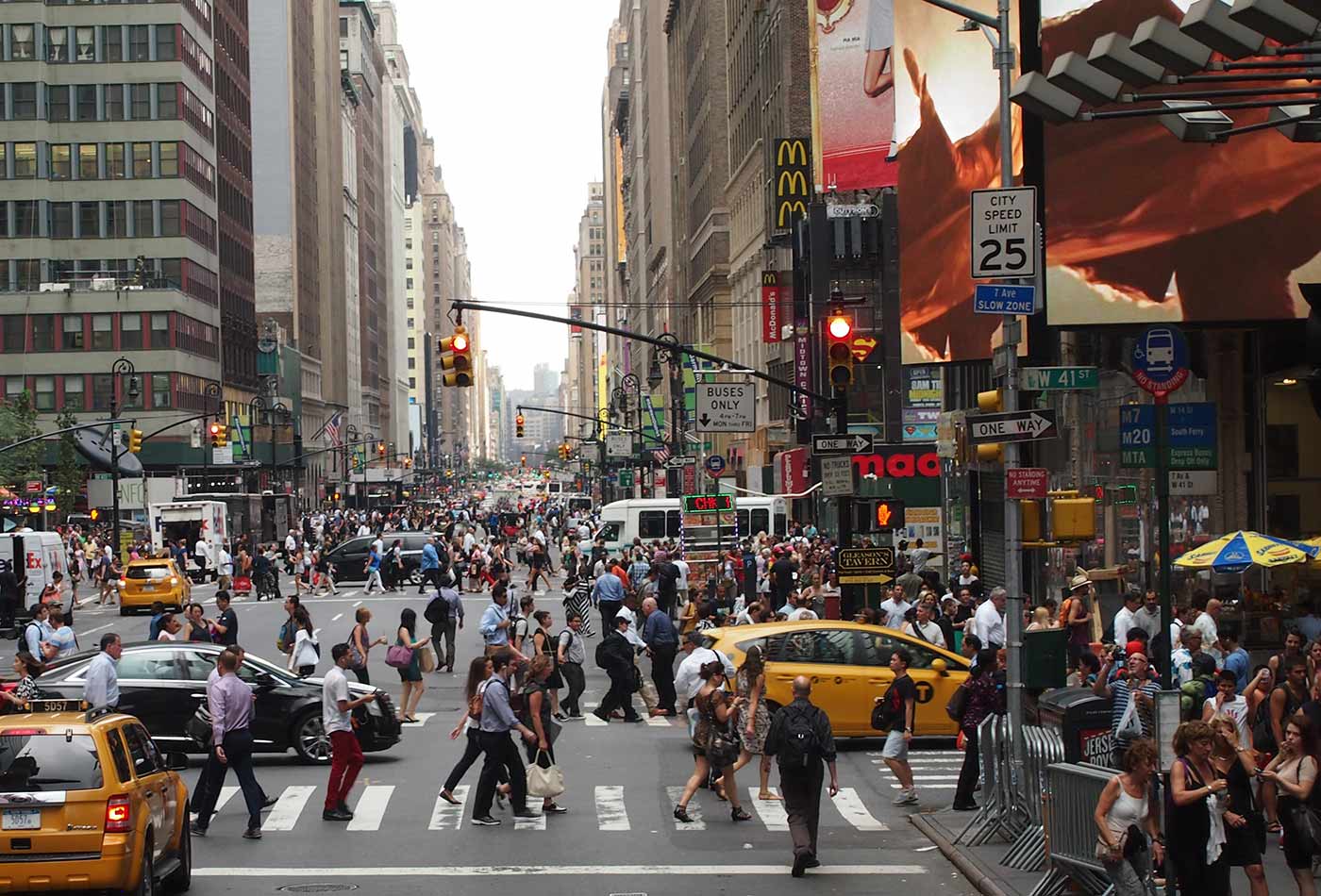
We’re in an empirical era of machine learning. Companies are now building platforms that facilitate experimentation and collaboration. At our upcoming Strata Data Conference in San Jose, we have many tutorials and sessions on “Data Science and Machine Learning” (including two days of sessions on enterprise applications of deep learning), and “Data Engineering & Architecture” (including sessions on streaming/real-time from several open source communities). If you want to understand how companies are using big data and machine learning to reinvigorate their businesses, there are many case studies on the schedule geared toward hands-on technologists, and sessions aimed at managers and executives.
Putting data and machine learning technologies to work
Over the past few years, companies have invested in data gathering and data management technologies, and many have began unlocking value from their vast repositories. From the inception of Strata, we’ve featured case studies from companies across a wide variety of industries. This marks the second year we will offer a series of executive briefings over two days. These are 40-minute overviews on important topics in big data and data science, aimed at executives and managers tasked with understanding how to incorporate these technologies and techniques into their business operations. Topics will include privacy and security, AI and machine learning, data infrastructure, and culture (including hiring, managing, and nurturing data teams). We also have tutorials and many case studies tailored for managers and executives, including a day-long focus on media and ad tech.
Data and machine learning are becoming more pervasive—and a greater competitive differentiator
As the use of data technologies and analytics become more prevalent, it’s critical to keep up with the latest technologies, architectures, best practices, and methodologies. The increasing importance of data and machine learning means that managers and analysts also need to familiarize themselves with critical tools and technologies. We’ve assembled a series of two-day training courses for engineers and developers, data scientists and analysts, and managers. We’re expanding our suite of courses to cover critical technologies and concepts for a broad set of workers:
- Data science for managers
- Apache Spark programming
- Data science and machine learning with Apache Spark
- Machine learning with TensorFlow
- Real-time systems with Spark Streaming and Kafka
- Machine learning with PyTorch
- Hands-on data science with Python
The importance of data pipelines and data integration
Media coverage of machine learning exploded last year. But anyone who works with machine learning will tell you that (at least for now), everything depends on having large (labeled) data sets. Data used for analytics and in machine learning products typically come from a variety of sources. There are usually a series of steps to combine, validate, and prepare data for use in machine learning. For many data scientists and machine learning engineers, maintaining robust data pipelines remains a critical part of their jobs. We’ve assembled a series of sessions to showcase some of the current best practices for building scalable data pipelines:
- The future of ETL isn’t what it used to be
- Radically modular data ingestion APIs in Apache Beam
- How to build leakproof stream processing pipelines with Apache Kafka and Apache Spark
- Semi-automated analytic pipeline creation and validation using active learning
- Building a flexible ML pipeline at a B2B AI startup
- Pipeline testing with Great Expectations
Graphs and time series
Graphs and time series were there from the outset of the renewed interest in distributed data management systems and other big data technologies. Many problems and use cases — including fraud detection — lend themselves to graph or time series technologies and methods. This past year, a new generation of technologies and methods (including deep learning) have injected excitement into both graphs and time series. The sessions in San Jose will cover data storage and management, analytics and machine learning, and real-world applications of graphs and time series.
- Graphs and Time-series sessions at Strata Data San Jose