Welcome to Intelligence Matters
Casting a critical eye on the exciting developments in the world of AI.
Editor’s note: this post was co-authored by Ben Lorica and Roger Magoulas. It’s the kick-off to our Intelligence Matter series.
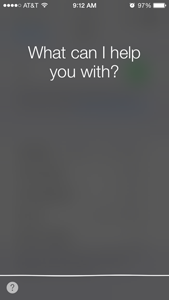
Today we’re kicking off Intelligence Matters (IM), a new series exploring current issues in artificial intelligence, including the connection between artificial intelligence, human intelligence and the brain. IM offers a thoughtful take on recent developments, including a critical, and sometimes skeptical, view when necessary.
True AI has been “just around the corner” for 60 years, so why should O’Reilly start covering AI in a big way now? As computing power catches up to scientific and engineering ambitions, and as our ability to learn directly from sensory signals — i.e., big data — increases, intelligent systems are having a real and widespread impact. Every Internet user benefits from these systems today — they sort our email, plan our journeys, answer our questions, and protect us from fraudsters. And, with the Internet of Things, these system have already started to keep our houses and offices comfortable and well-lit, our data centers running more efficiently, our industrial processes humming, and even are driving our cars.
Of course, these systems don’t exist in a vacuum; in fact, some of the most fascinating aspects of machine intelligence arise from their deep interconnections with other technologies. The impact of big data and the Internet of Things will both be magnified once these massive information streams can be interpreted and acted upon by truly intelligent systems.
Semantics matter as well: artificial intelligence and related labels (machine learning, strong or true AI, artificial general intelligence) refer to a wide range of systems built with varied goals, technologies, and even philosophical foundations. In addition, attitudes associated with “AI” have shifted radically over time, from wild optimism to skepticism, and even deep stigma. We plan on disentangling these definitional issues in at least one upcoming post.
AI has been through several hype cycles, but after years of being pushed into the shadows in favor of more limited and domain-specific machine learning efforts, there is a new boom in frankly — and unapologetically — ambitious AI projects at companies like Google, Facebook, and IBM, as well as research organizations like the Allen Institute for Artificial Intelligence. Researchers finally have the compute power to aim high, confidence won from recent advances, and resources from the explosive growth of the tech industry. These AI efforts are key to these companies’ long-term growth and competitiveness.
Some see this commercial vision as naive, shortsighted and limited — some very smart folks argue that real AI could be our last invention. But is this singularity scenario realistic, whether the resulting intelligences are friendly or not? More generally, how are forecasts led astray when we extrapolate from present trends — for instance, the supposedly inevitable consequences of exponentially-increasing compute power.
With two sides — marketing boosterism and the doomsayers — both vying for mindshare, it’s hard for journalists and other interested observers to interpret new developments. It’s also hard to evaluate claims of progress on their merits; the history of AI is full of approaches that showed early promise, only to turn out as blind alleys and false summits. In fact, Facebook’s AI lab director and neural network pioneer Yann Lecun compares AI research to “…driving in a thick fog and we don’t realize that our highway is really a parking lot with a brick wall at the far end.” Many smart people have made that mistake, and every new wave in AI was followed by a period of unbounded optimism, irrational hype, and a backlash. We likely won’t know which approach succeeds in creating “true” AI until we see it.
With these difficulties firmly in mind, we strive to place new developments in context, including turning a critical eye when appropriate. The field is moving fast, and there is no shortage of genuinely exciting progress, along with misguided endeavors, naked opportunism, and even deliberate bubble inflation.
Some questions we’ll explore:
- What is a good definition of AI for the current day? And what is the best way to define intelligence itself, in both its natural and synthetic forms?
- How can we disentangle the varied aims of different AI projects — from building valuable new products to understanding the mysteries of the human mind to creating wholly new forms of superintelligence?
- How much does an understanding of the brain matter in creating intelligent systems? And what does it mean for a system to work “like the brain”?
- How are the machine learning techniques that are increasingly being used by companies to understand and act on big data related to artificial intelligence?
- Do systems like Siri and Watson represent major advances toward the ultimate goals of AI?
- What is the relationship between AI systems and crowdsourced intelligence? Which problems are best suited for each approach?
- What is deep learning, and why are so many companies excited about it? Will deep learning get us to true AI?
Beau Cronin will serve as lead correspondent of Intelligence Matters
Over the last few years, we’ve had some deep discussions about probabilistic programming and AI with our friend Beau Cronin. These discussions coalesced into the notion of a new series covering AI topics, and we’re thrilled that Cronin can serve as lead correspondent for Intelligence Matters.
Beau has both an academic background and a wealth of practical experience in the AI field, combined with a deep curiosity and a keen desire to share his knowledge. He holds a doctorate in computational neuroscience from MIT and co-founded two startups that used probabilistic inference. We’re excited to have Beau on board to cover the exciting world of AI and other intelligence matters, and we invite you to participate: please join the discussion in the comments section or by connecting with Beau on Twitter.