What Does Copyright Say about Generative Models?
Not much.
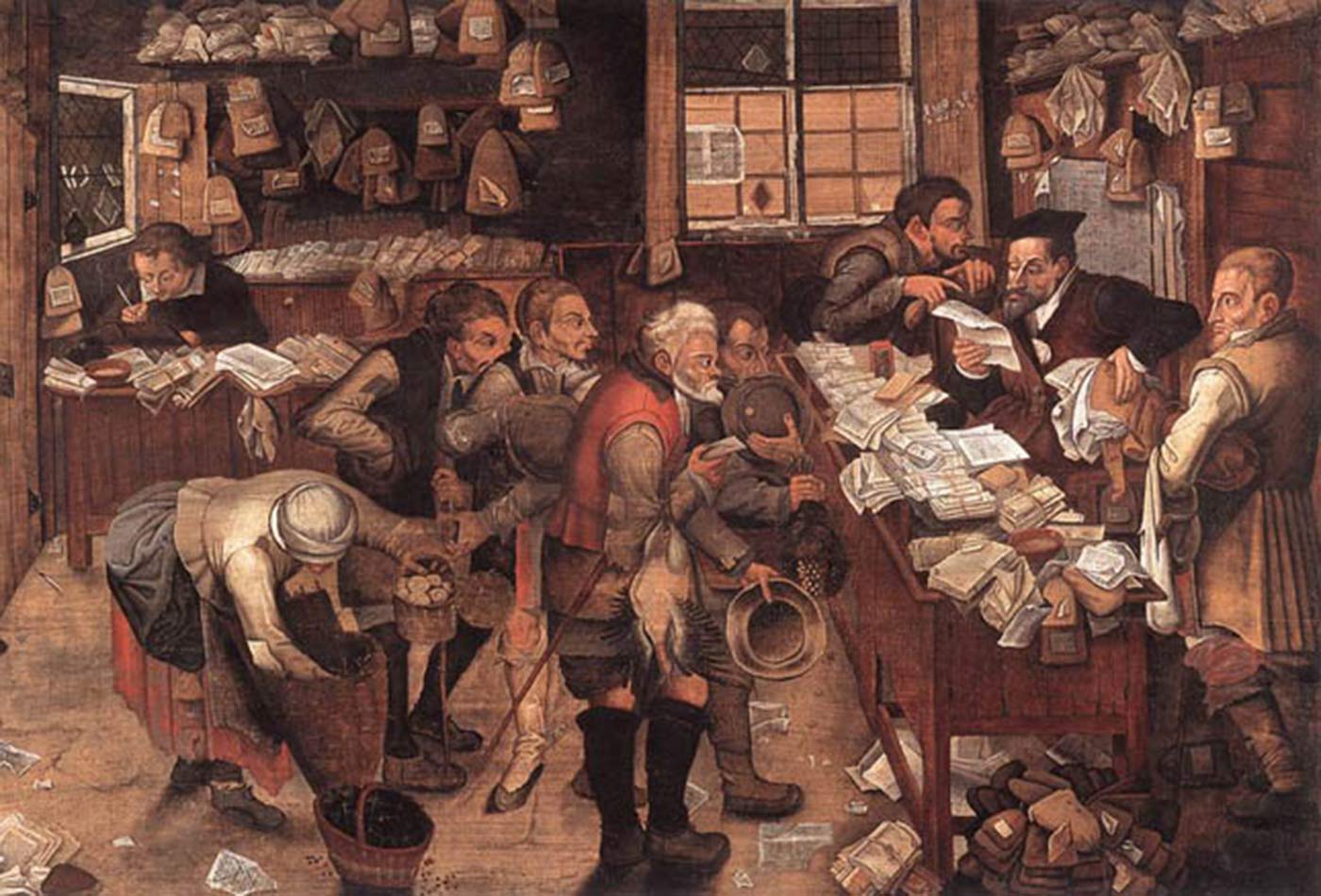
The current generation of flashy AI applications, ranging from GitHub Copilot to Stable Diffusion, raise fundamental issues with copyright law. I am not an attorney, but these issues need to be addressed–at least within the culture that surrounds the use of these models, if not the legal system itself.
Copyright protects outputs of creative processes, not inputs. You can copyright a work you produced, whether that’s a computer program, a literary work, music, or an image. There is a concept of “fair use” that’s most applicable to text, but still applicable in other domains. The problem with fair use is that it is never precisely defined. The US Copyright Office’s statement about fair use is a model for vagueness:
Under the fair use doctrine of the U.S. copyright statute, it is permissible to use limited portions of a work including quotes, for purposes such as commentary, criticism, news reporting, and scholarly reports. There are no legal rules permitting the use of a specific number of words, a certain number of musical notes, or percentage of a work. Whether a particular use qualifies as fair use depends on all the circumstances.
We are left with a web of conventions and traditions. You can’t quote another work in its entirety without permission. For a long time, it was considered acceptable to quote up to 400 words without permission, though that “rule” was no more than an urban legend, and never part of copyright law. Counting words never shielded you from infringement claims–and in any case, it applies poorly to software as well as works that aren’t written text. Elsewhere the US copyright office states that fair use includes ”transformative” use, though “transformative” has never been defined precisely. It also states that copyright does not extend to ideas or facts, only to particular expressions of those facts–but we have to ask where the “idea” ends and where the “expression” begins. Interpretation of these principles will have to come from the courts, and the body of US case law on software copyright is surprisingly small–only 13 cases, according to the copyright office’s search engine. Although the body of case law for music and other art forms is larger, it’s even less clear how these ideas apply. Just as quoting a poem in its entirety is a copyright violation, you can’t reproduce images in their entirety without permission. But how much of a song or a painting can you reproduce? Counting words isn’t just ill-defined, it is useless for works that aren’t made of words.
These rules of thumb are clearly about outputs, rather than inputs: again, the ideas that go into an article aren’t protected, just the words. That’s where generative models present problems. Under some circumstances, output from Copilot may contain, verbatim, lines from copyrighted code. The legal system has tools to handle this case, even if those tools are imprecise. Microsoft is currently being sued for “software piracy” because of GitHub. The case is based on outputs: code generated by Copilot that reproduces code in its training set, but that doesn’t carry license notices or attribution. It’s about Copilot’s compliance with the license attached to the original software. However, that lawsuit doesn’t address the more important question. Copilot itself is a commercial product that is built a body of training data, even though it is completely different from that data. It’s clearly “transformative.” In any AI application, the training data is at least as important to the final product as the algorithms, if not more important. Should the rights of the authors of the training data be taken into account when a model is built from their work, even if the model never reproduces their work verbatim? Copyright does not adequately address the inputs to the algorithm at all.
We can ask similar questions about works of art. Andy Baio has a great discussion of an artist, Hollie Mengert, whose work was used to train a specialized version of Stable Diffusion. This model enables anyone to produce Mengert-like artworks from a textual prompt. They’re not actual reproductions; and they’re not as good as her genuine artworks–but arguably “good enough” for most purposes. (If you ask Stable Diffusion to generate “Mona Lisa in the style of DaVinci,” you get something that clearly looks like Mona Lisa, but that would embarrass poor Leonardo.) However, users of a model can produce dozens, or hundreds, of works in the time Mengert takes to make one. We certainly have to ask what it does to the value of Mengert’s art. Does copyright law protect “in the style of”? I don’t think anyone knows. Legal arguments over whether works generated by the model are “transformative” would be expensive, possibly endless, and likely pointless. (One hallmark of law in the US is that cases are almost always decided by people who aren’t experts. The Grotesque Legacy of Music as Property shows how this applies to music.) And copyright law doesn’t protect the inputs to a creative process, whether that creative process is human or cybernetic. Should it? As humans, we are always learning from the work of others; “standing on the shoulders of giants” is a quote with a history that goes well before Isaac Newton used it. Are machines also allowed to stand on the shoulders of giants?
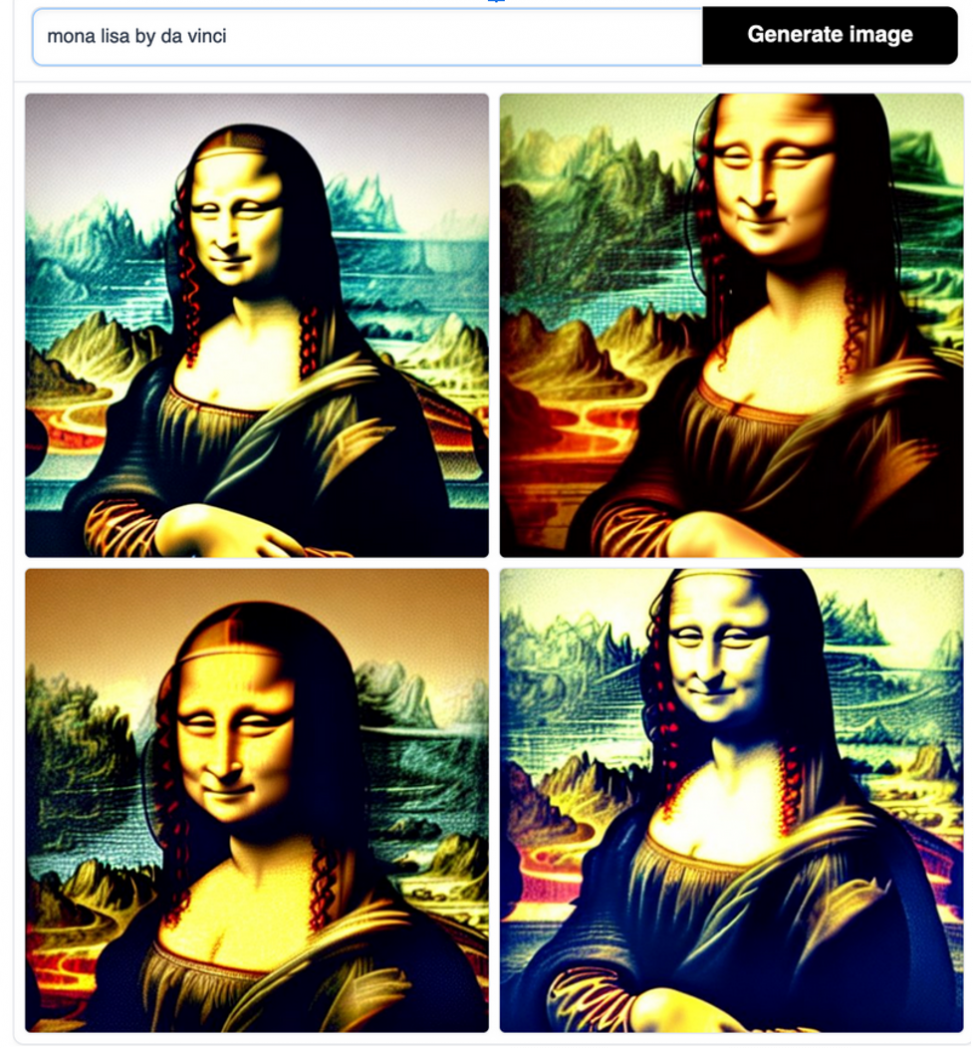
Mona Lisa in the style of DaVinci. DaVinci isn’t worried. (Courtesy Hugo Bowne-Anderson)
To think about this, we need an understanding of what copyright does culturally. It’s a double-edged sword. I’ve written several times about how Beethoven and Bach made use of popular tunes in their music, in ways that certainly wouldn’t be legal under current copyright law. Jazz is full of artists quoting, copying, and expanding on each other. So is classical music–we’ve just learned to ignore that part of the tradition. Beethoven, Bach, and Mozart could easily have been sued for their appropriation of popular music (for that matter, they could have sued each other, and been sued by many of their “legitimate” contemporaries)–but that process of appropriating and moving beyond is a crucial part of how art works.
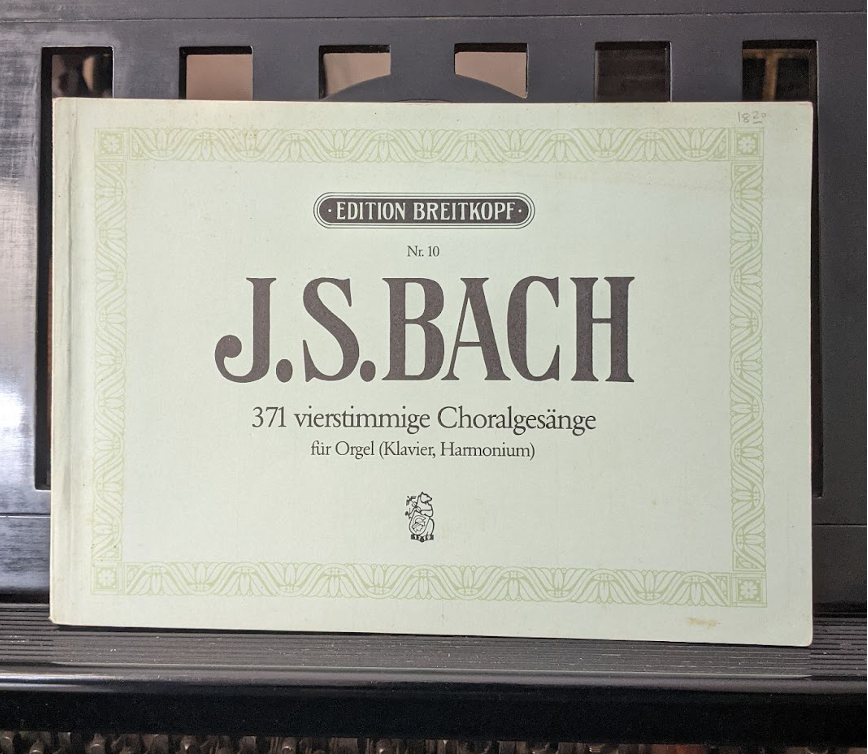
J. S. Bach’s 371 Choral Copyright Violations. He would have been in trouble if copyright as we now understand it had existed.
We also have to recognize the protection that copyright gives to artists. We lost most of Elizabethan theater because there was no copyright. Plays were the property of the theater companies (and playwrights were often members of those companies), but that property wasn’t protected; there was nothing to prevent another company from performing your play. Consequently, playwrights had no interest in publishing their plays. The scripts were, literally, trade secrets. We’ve probably lost at least one play by Shakespeare (there’s evidence he wrote a play called Love’s Labors Won); we’ve lost all but one of the plays of Thomas Kyd; and there are other playwrights known through playbills, reviews, and other references for whom there are no surviving works. Christopher Marlowe’s Doctor Faustus, the most important pre-Shakespearian play, is known to us through two editions, both published after Marlowe’s death, and one of those editions is roughly a third longer than the other. What did Marlowe actually write? We’ll never know. Without some kind of protection, authors had no interest in publishing at all, let alone publishing accurate texts.
So there’s a finely tuned balance to copyright, which we almost certainly haven’t achieved in practice. It needs to protect creativity without destroying the ability to learn from and modify earlier works. Free and open source software couldn’t exist without the protection of copyright–though without that protection, open source might not be needed. Patents were intended to play a similar role: to encourage the spread of information by guaranteeing that inventors could profit from their invention, limiting the need for “trade secrets.”
Copying works of art has always been (and still is) a part of an artist’s education. Authors write and rewrite each other’s works constantly; whole careers have been made tracing the interactions between John Milton and William Blake. Whether we’re talking about prose or painting, generative AI devalues traditional artistic technique (as I’ve argued), though possibly giving rise to a different kind of technique: the technique of writing prompts that tell the machine what to create. That’s a task that is neither simple nor uncreative. To take Mona Lisa and go a step further than Da Vinci–or to go beyond facile imitations of Hollie Mengert–requires an understanding of what this new medium can do, and how to control it. Part of Google’s AI strategy appears to be building tools that help artists to collaborate with AI systems; their goal is to enable authors to create works that are transformative, that do more than simply reproducing a style or piecing together sentences. This kind of work certainly raises questions of reproducibility: given the output of an AI system, can that output be recreated or modified in predictable ways? And it might cause us to realize that the old cliche “A picture is worth a thousand words” significantly underestimates the number of words it takes to describe a picture.
How do we best protect creative freedom? Is a work of art something that can be “owned,” and what does that mean in an age when digital works can be reproduced perfectly, at will? We need to protect both the original artists, like Hollie Mengert, and those who use their original work as a springboard to go beyond. Our current copyright system does that poorly, if at all. (And the existence of patent trolls demonstrates that patent law hasn’t done much better.) What was originally intended to protect artists has turned into a rent-seeking game in which artists who can afford lawyers monetize the creativity of artists who can’t. Copyright needs to protect the input side of any generative system: it needs to govern the use of intellectual property as training data for machines. But copyright also needs to protect the people who are being genuinely creative with those machines: not just making more works “in the style of,” but treating AI as a new artistic medium. The finely tuned balance that copyright needs to maintain has just become more difficult.
There may be solutions outside of the copyright system. Shutterstock, which previously announced that they were removing all AI-generated images from their catalog, has announced a collaboration with OpenAI that allow the creation of images using a model that has only been trained on images licensed to Shutterstock. Creators of the images used for training will receive a royalty based on images created by the model. Shutterstock hasn’t released any details about the compensation plan, and it’s easy to suspect that the actual payments will be similar to the royalties musicians get from streaming services: microcents per use. But their approach could work with the right compensation plan. Deviant Art has released DreamUp, a model based on Stable Diffusion that allows artists to specify whether models can be trained on their content, along with identifying all of its outputs as computer generated. Adobe has just announced their own set of guidelines for submitting generative art to their Adobe Stock collection, which requiring that AI-generated art be labeled as such, and that the (human) creators have obtained all the licenses that might be required for the work.
These solutions could be taken a step further. What if the models were trained on licenses, in addition to the original works themselves? It is easy to imagine an AI system that has been trained on the (many) Open Source and Creative Commons licenses. A user could specify what license terms were acceptable, and the system would generate appropriate output–including licenses and attributions, and taking care of compensation where necessary. We need to remember that few of the current generative AI tools that now exist can be used “for free.” They generate income, and that income can be used to compensate creators.
Ultimately we need both solutions: fixing copyright law to accommodate works used to train AI systems, and developing AI systems that respect the rights of the people who made the works on which their models were trained. One can’t happen without the other.