Decision-Making in a Time of Crisis
A Bad Outcome Doesn't Mean a Bad Decision
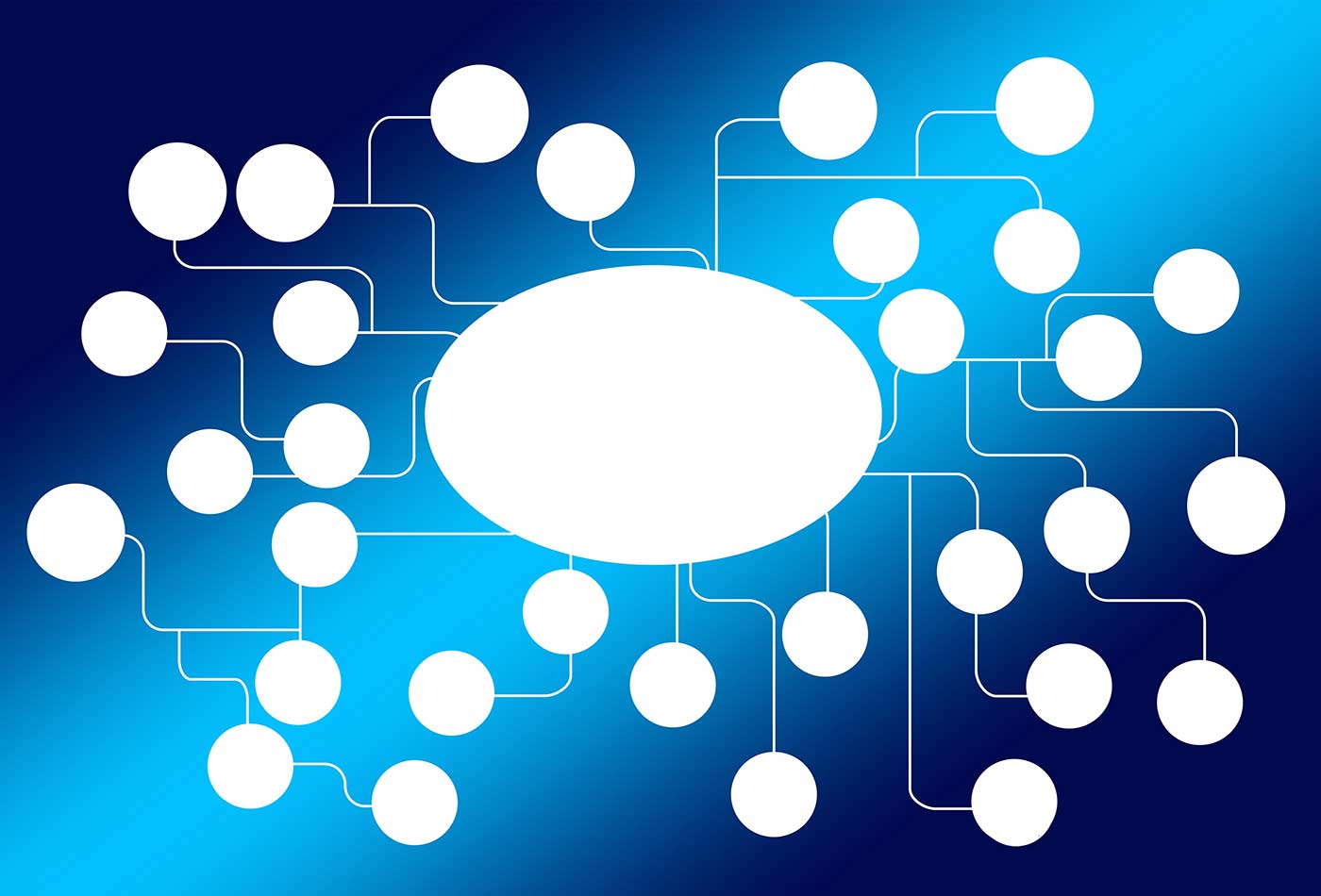
In the 1996 cult classic film Swingers, two friends, Trent and Mike (played by Vince Vaughan and Jon Favreau, respectively) make an impromptu trip to Las Vegas. At the blackjack table, Mike gets dealt an 11 and Trent tells him to double down. Mike responds “What?!” and Trent replies “Double down, baby. You gotta double down on an eleven.” Mike doubles down and loses the hand. The next scene opens with:
Trent: I’m telling you, baby, you always double down on an eleven.
Mike: Yeah? Well obviously not always!
Trent: Always, baby.
Mike: I’m just saying, not in this particular case.
Trent: Always.
Mike: But I lost! How can you say always?!?
Mike had made the common error of equating a bad outcome with a bad decision. The decision-making process was fine. We know, statistically, that doubling down on an 11 is a good (and common) strategy in blackjack. But when making a decision under uncertainty about the future, two things dictate the outcome: (1) the quality of the decision and (2) chance. The quality of the decision is based on known information and an informed risk assessment, while chance involves hidden information and the stochasticity of the world. The latter resulted in Mike losing his blackjack hand. It was luck, not the decision to double down.
We currently have a lot of serious and challenging decisions to make at personal, societal, and global levels and none of them are as simple as a game of blackjack. This essay is about how to take a more principled approach to making decisions under uncertainty and aims to provide certain conceptual and cognitive tools for how to do so, not what decisions to make. These tools include how to:
- Think probabilistically and understand the nature of predictions.
- Consider risk not only in terms of likelihood but also in terms of the impact of your decisions.
- Interrogate reported data and information with a healthy skepticism through thinking about the processes that generate the data.
- Prioritize which decisions to make and what actions to take in an uncertain world.
There are two key differences between the type of decisions we need to make currently and Mike’s decision to double down in blackjack. Firstly, in cases such as re-opening economies, the decision space isn’t binary—it’s not “re-open the economy or not,” it’s “how? how much? when? and how do we know when to reel it back in?” Secondly, we know the odds in blackjack—it will take you some time, but you can write down a table of all the probabilities. When we know the probabilities of the important variables but don’t know the outcomes, it’s called risk. When we don’t know the probabilities, or even what all the important variables are, it’s called uncertainty. Recognizing the difference between risk and uncertainty is essential to knowing when you can and cannot completely calculate and assess risk. Thinking how to assess decision quality, as opposed to outcome quality, in both cases is key, though, as Swingers makes clear.
Thinking in Bets, Annie Duke’s 2018 book about making decisions under uncertainty, has many analogous examples operating under both risk and uncertainty, such as the infamous 2015 Super Bowl XLIX Seahawks’ decision to pass the ball in the final 26 seconds. The pass was intercepted, the Seahawks lost, and we saw countless headlines such as “Dumbest Call in Super Bowl History Could Be Beginning of the End for Seattle Seahawks” and “Seahawks Lost Because of the Worst Call in Super Bowl History.” As Duke astutely points out, agreeing with several commentators such as FiveThirtyEight’s Benjamin Morris and Slate’s Brian Burke, the decision to pass was eminently defensible, as “in the previous fifteen seasons, the interception rate in that situation was about 2%.” Tellingly, when Duke asks business executives to write down their best and worst decisions of the past year, they invariably write down the best and worst outcomes. It is all too human to judge decisions by their outcomes. Duke refers to this as resulting. We need to rationally decouple decision quality from outcome quality. One challenge is that we are evaluated on outcomes, not decisions, for the most part: a Chief Sales Officer is evaluated on deals closed and annual recurring revenue, not the decisions they make, per se. The success of a company is likewise determined by outcome quality, not decision quality. However, as with blackjack, if we are to evaluate decision making by looking at outcomes, it is more productive to look at the long run frequencies of good and bad outcomes to evaluate both the decision and the strategy that led to the decision. In the long run, the fluctuations of chance will average out.
Another key barrier to rationally evaluating decision quality is that we are not adept at dealing with uncertainty and thinking probabilistically. We saw this after the 2016 U.S. Presidential election when people said the pollsters’ predictions were wrong, because they had Clinton as the front-runner. But a prediction that Clinton had a 90% chance of winning was not an incorrect prediction, even when Trump won: Trump winning was merely that 10% chance playing out in reality. The statement “the prediction was wrong” is assessing the quality of the prediction based on the outcome, committing the same error as assessing the quality of a decision based on outcome: it’s resulting. For this reason, let’s drill down a bit into how bad we really are at thinking probabilistically and dealing with uncertainty. To do so, let’s stick with the example of the 2016 U.S. election.
Making Predictions and Thinking Probabilistically
Many intelligent people were surprised when Donald Trump won the presidency, even though FiveThiryEight gave him a 29% chance of winning. Allen Downey, Professor at Olin College, points out that a 29% chance is more likely than seeing two heads when flipping two coins (25% chance), an occurrence that wouldn’t surprise any of us. Even if we believed the forecasts that gave Trump a 10% chance of winning, this is just slightly less likely than seeing three heads in three coin tosses (12.5%), which also would not surprise many people. Consider a 10% likelihood in this way: “would you board a plane if the pilot told you it had a 90% chance of landing successfully?”, as Nate Silver asks in The Signal and the Noise.
Why are we so bad at interpreting probabilistic predictions, such as the probability of Trump winning the presidency? One possibility, suggested by Downey, is that we generally interpret probabilistic predictions as deterministic predictions with a particular degree of certainty. For example, “Clinton has a 90% chance of winning” would be interpreted as “The poll says Clinton will win and we’re 90% sure of this.” As Downey says, “If you think the outcome means that the prediction was wrong, that suggests you are treating the prediction as deterministic.”
Forecasters and pollsters are aware of this deep challenge. Nate Silver and FiveThirtyEight have put substantial thought into how to report their probabilistic forecasts. In the 2018 midterms, for example, they began to make forecasts such as “1 in 5 chance Democrats win control (19.1%); 4 in 5 chance Republicans keep control (80.9%),” which is careful to express the probabilistic nature of the prediction. I recalled this mindful use of language when I recently had a COVID-19 test and the doctor reported “the test did not detect the presence of COVID-19,” instead of “the test came back negative.” Language is important, particularly in situations where our intuition doesn’t work well, such as in probabilistic forecasts and data reporting. So knowing that we need to make sure we judge decision and prediction quality based on what was known at the time of decision or prediction, respectively, how do we go about thinking through the risks to make decisions in the first place?
Risk, Probability, Impact, and Decisions
I’ve had many discussions around risk assessment and decision-making with respect to COVID-19, as we likely all have recently. One common and concerning throughline is that many people appear to make risk assessments based on likelihood without considering impact. For example, in different conversations, I told several friends that my COVID-19 test had come back negative. Each friend replied along similar lines, saying that it meant that I could visit my parents, who are both in high risk groups. Ignoring the false negative rate, I replied that it would still be possible for me to pick up COVID-19 after the test and take it into their house, and my friends’ responses were all “but it is soooo unlikely.” This may be true, but the downside risk in this case could be fatal. When making decisions under uncertainty, it is a mistake to consider likelihood alone: you need to consider impact.
For example, let’s say there’s a burger that you’ve heard is great and you really want to try it. If there’s a 20% chance that it will give you some mild stomach trouble (possible but low impact), perhaps you’ll still try it. If there’s a 0.1% (1 in 1,000) chance that it will kill you (very unlikely but high impact), I’d be surprised and/or concerned if you decided to eat it, after assessing the risk.
This example, although a bit silly (and perhaps delicious), has many elements of what you need to make decisions under uncertainty: consideration of likelihood of different potential outcomes, upside risk (enjoying a delicious burger), and downside risk (stomach trouble and dying, respectively).
Now imagine a different scenario. Instead of eating a burger, we’re talking about surgery to cure a painful but not life-threatening condition, spine surgery, for example, and there is a 0.1% chance of death. The downside risk is the same, fatal, but the upside risk is a lot more impactful than eating a burger, so there’s an increased chance of you taking on the downside risk.
Instead of viewing a risk assessment along the sole axis of likelihood, we also have to consider impact. One useful tool for doing this is known as a risk matrix (common in business settings), a table that has axes likelihood and impact:
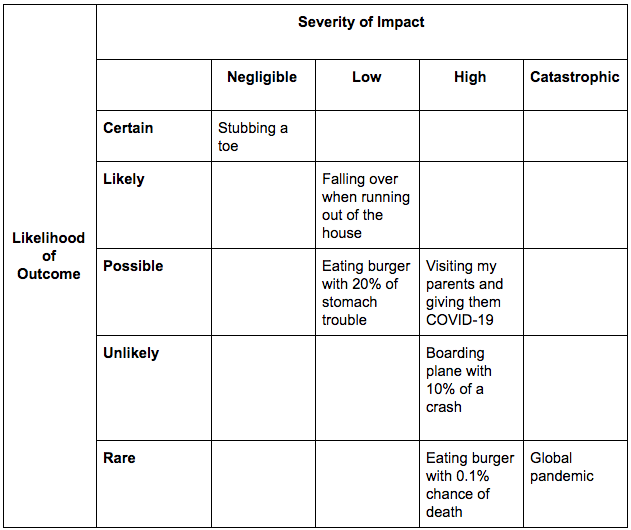
This risk matrix captures the likelihood of outcome on the vertical axis and the impact on the horizontal axis and is based on a risk matrix from the Wikipedia page. It references decisions described in the main body of the essay and it does not describe the upside risk in these situations (which would require a third dimension!)
Deciding whether to wear a mask outside is a current example. There are multiple personal and societal risks to consider: wearing a mask reduces the transmission of COVID-19 (upside risk; particularly important given the risk of being an asymptomatic transmission vector) but if we all go out and panic buy PPE masks, there will be a devastating lack of supply for frontline healthcare workers (downside risk; note that the risk is not for us, but for frontline healthcare workers and, by extension, society, so in this case we’re thinking about making individual decisions based around societal, not only personal, risk). Once you realize that we can all avoid the downside risk by making masks from household items or buying cotton masks online from dressmakers and shirtmakers, the decision to wear a mask is a no-brainer.
This example also illustrates how the decision space can be a lot larger than originally envisioned: the choice is not merely between “wearing a mask that a doctor or nurse will need” or “not.” There are always more options than are first apparent. Our work is to find the ones that minimize risk. We saw this play out as the CDC and many governments went from recommending only people who have symptoms wear masks to recommending that everybody wear masks.
This is a guide for how to think about making decisions, not what decisions to make. The decisions any individual makes are also a function of how risk-friendly and risk-averse that individual is. Financial advisors are known to provide questionnaires to determine where their clients lie on the risk friendliness-aversion spectrum and advise accordingly. I am generally risk friendly but, when it comes to a global pandemic and matters of life and death, I am highly risk averse. I would encourage you to be also, and remind you that your actions impact potentially a huge number of people, even if you are in a low risk group and not particularly concerned about your own health.
At a far larger scale of decision-making, governments need to make decisions around when and how to re-open economies. They need to consider a number of things. In particular, the fact that we have a public health crisis and a resulting economic crisis, which feeds back into the public health crisis, along with creating its own health crises, which economic downturns are known to. Ideally, we could re-open the economy to an extent that will not exacerbate the COVID-19 crisis but enough to reduce the economic crisis and all the downstream effects. This is once again opening up the decision space: it isn’t “re-open the economy or not”; it’s figuring out when to and by how much.
Figuring out likelihoods and impact of all our governmental decisions is incredibly challenging work. It’s the same on a personal level. We need to consider both the likelihood of outcomes resulting from our different decisions, along with their impact, but how can we actually do this? Having good quality information is key, as is knowing what our blind spots are, that is, knowing what we don’t know. So let’s now dive into thinking about the quality of the data we’re seeing every day, and what type of information and knowledge we can extract from it.
Data, Information, Knowledge, and Decision-Making
One of the most important steps in acknowledging what our blind spots are is knowing the limitations of the data and information that we receive.
For example, when we see a chart of the number of reported cases of COVID-19 over time, it is natural and tempting to think of this as a proxy for the evolution of the number of actual cases. I’ve heard rational humans make statements such as “it may not be quite right, but it’s all we have and probably captures the trend.” But it may not even do that. The number of reported cases is a function of many things, including the number of tests available, the willingness of people to be tested, the willingness of any particular government to report their findings, and a time lag resulting from the COVID-19 incubation period. In terms of government incentives to report their findings, it is key to keep front of mind that the reporting of a COVID death is a political and politicized act. There has been huge skepticism of official counts coming out of China and, as we re-open cities across the world, governments will be incentivized to under-report cases, both to justify the decisions to re-open and in the name of protecting economies.
In terms of the number of reported cases being a function of the number of available tests, take this extreme limit case: one day, there are no tests, so no reported cases, and the next day there are a huge number of tests; in this case, even if there were a decrease in the total number of actual cases, a huge spike would be reported.
As a real-world example, Nate Silver reported:
Washington State is a good example of the importance of accounting for the number of tests when reporting COVID-19 case counts. Remember I mentioned a couple of days ago how their number of cases in WA had begun to stabilize? Well, guess what happened… Today, they reported 189 positives, along with 175 yesterday, as compared with an average of 106 positives per day in the 7 days before that. So, not great on the surface… new cases increased by 70%! But you also have to look at the number of tests. Washington conducted 3,607 tests today and 2,976 yesterday. By comparison, they’d conducted an average of 1,670 tests in the 7 days before that. So they’ve increased testing capacity by 97% over their baseline. Meanwhile, detected cases have increased, but by “only” 70%. Looked at another way: Today, 5.2% of Washington’s tests came up with a positive result. Yesterday, 5.9% did. In the 7 days before that, 6.4% of them did. So, there *is* a bit of progress after all. Their number of new positives *as a share of new tests* is slightly declining. For the time being, 1) the large (perhaps very large) majority of coronavirus positives are undetected and 2) test capacity is ramping up at extremely fast rates, far faster than coronavirus itself would spread even under worst-case assumptions. So long as those two things hold, the rate of increase in the number of *detected* cases is primarily a function of the rate of increase in the number of *tests* and does not tell us that much about how fast the actual *infection* is spreading.
Silver went on to write an article entitled “Coronavirus Case Counts Are Meaningless” with a subtitle “Unless you know something about testing. And even then, it gets complicated.”
In a similar manner, the number of reported deaths is also likely to be a serious underestimate, because, in many places, to be a reported COVID-19 death, you need to be tested and diagnosed. Bloomberg reports that, in reference to Italy, “many will die in their houses or nursing homes and may not even be counted as COVID-19 cases unless they’re tested post-mortem.” As Dr. Anthony Fauci, one of the top US government infectious disease experts and member of 45’s COVID-19 task force, stated, “there may have been people who died at home who did have COVID, who are not counted as COVID because they never really got to the hospital.” It is important to stress that this undercounting will disproportionately impact demographics that have less wealth and less access to healthcare, including those already structurally oppressed, such as people of color. One way to correct for this bias in the data is to look at the statistics of “excess deaths,” the numbers when compared with previous years.
A conceptual tool that I like to use when thinking about these types of biases in the data collection and data reporting processes is Wittgenstein’s Ruler, as introduced by essayist, statistician, and professional provocateur Nassim Nicholas Taleb in Fooled By Randomness:
Unless you have confidence in the ruler’s reliability, if you use a ruler to measure a table you may also be using the table to measure the ruler.
The first concept here is that, if your measurement device is broken, whether it be a ruler or a pandemic testing system, it’s not telling you anything of value about the real world. Worse, it may be providing incorrect information. The second concept is that, if you can find out something about the length of the table by other means, you may be able to infer properties of the ruler. In our current case, this could mean that if we knew more about actual death rate (by, for example, considering the statistics of “excess deaths”), we could infer the flaws in our reported deaths data collection, analysis, and reporting processes.1
Moreover, data collection and data reporting are political acts and processes embedded in societies with asymmetric power relations, and most often processes controlled by those in positions of power. In the words of Catherine D’Ignazio and Lauren F. Klein in Data Feminism, “governments and corporations have long employed data and statistics as management techniques to preserve and unequal status quo.” It is a revelation to realize that the etymology of the word statistics comes from the term statecraft2 and the ability of states and governments to wield power through the control of data collection and data reporting (they decide what is collected, reported, how it is reported, and what decisions are made).
The major takeaway from this section is to approach reported data with an educated skepticism, recognize the potential biases in reported data, and realize that there is a huge amount of uncertainty here. Easier said than done, of course, particularly when we live in a world of information glut and the sheer number of decisions we need to make seems to increase daily. So how do we actually think about incorporating information into our decision-making processes? And how do we prioritize which decisions to make and actions to take?
Information Anxiety, Decision Fatigue, and the Scale of Things
In The Signal and the Noise, Nate Silver points out that we’re drowning in information and “we think we want information when we really want knowledge.” What we really now need is knowledge, which involves understanding, and an ability to incorporate this knowledge into our decision-making processes.
The number of decisions that a modern human has to make daily, consciously or otherwise, is staggering: estimates are around 35,000. Decision fatigue is real when one is faced with too many decisions, one after the other. Decision paralysis and the tyranny of choice3 are real, particularly in light of the vast swathes of content on display in the marketplace of the attention economy. This is why terms such as information anxiety, infobesity, and infoxication have evolved. And this was all pre-COVID-19.
Now we have a huge number of potentially fatal decisions to make and information to take in on so many scales:
- On the nanometre scale, the size of a coronavirus particle.
- On a microscale: “what did I just touch? Could I have picked up a particle?”
- On a bodily scale, when touching one’s nose by accident.
- On an apartment or house scale, when bringing deliveries or groceries in.
- On a family, professional, and small social network scale: “Who have I interacted with?”, “Who can I interact with?”
- On suburban, urban, state, national, and global scales: quarantine, lockdown, and shelter-in-place orders, supplies for hospitals, the closing of schools, shutting down the economy.
When contemplating the size of the universe in his Pensees, Blaise Pascal exclaimed “The eternal silence of these infinite spaces frightens me.” This would be a reasonable reaction to COVID-19, although one should also include an anxiety at the other end of the scale, the anxiety about the virus itself. From this perspective, as a global species, we’re stuck in the middle of a set of unforgiving scales that produce deep personal anxiety, global anxieties, and everything in between.
This is all to say that making decisions under uncertainty is tough and we’re not great at it, even under normal circumstances. During a global pandemic, it’s infinitely more difficult. We need to prioritize the decisions we want to make such as, for example, those involving the health of ourselves and those closest to us. When there are so many decisions to make, how do you go about ranking them, in terms of prioritization? A good heuristic here is to map out the space of possibilities resulting from your decisions, a practice called scenario planning, and prioritizing the ones that have the largest potential impact. In Thinking in Bets, Duke provides the example of After-School All-Stars (ASAS), a national non-profit she consulted. ASAS needed to prioritize the grants they were applying for. They had been prioritizing those that were worth the most, even if they were very unlikely to receive them. Duke proposed prioritizing the grants with highest expected value, that is, the total award grant multiplied by the estimated probability of receiving the grant (a grant X of $100,000 that they would win 10% of the time would be valued at $10,000; a grant Y of $50,000 that they would win 50% of the time would be valued at $25,000; in ASAS’ prioritization scheme, X would be prioritized, in Duke’s, Y would be: it’s worth less, but five times more likely). What Duke is implicitly performing in this calculus is scenario planning by looking at two possible futures (“awarded” or “declined” post-application) and averaging over them with respect to the probability of each. For more on scenario planning, I encourage you to check out Peter Schwartz’ book The Art of the Long View: Planning for the Future in an Uncertain World, along with Tim O’Reilly’s recent essay Welcome to the 21st Century: How To Plan For The Post-Covid Future, which “plays fast and loose with some of its ideas” (Tim’s words).
Most real-world cases are nowhere near as clean cut and consist of many cross-cutting decisions with varying levels of risk and uncertainty. However, taking a more principled approach to decision-making and prioritization by considering likelihood, impact, and scenario planning will improve decision quality. So will thinking more critically about risk, uncertainty, what the data we have actually means, and what information we really have about the world, as well as acknowledging our blind spots. In a word, making better decisions requires us to be more honest about uncertainty.
Footnotes
1 Taleb introduces the concept of Wittgenstein’s Ruler with respect to book reviews: “A book review, good or bad, can be far more descriptive of the reviewer than informational about the book itself.”
2 I discovered this fact from Chris Wiggins’ & Matt Jones’ course data: past, present, and future at Columbia University.
3 Also see The Paradox of Choice — Why Less is More, by Barry Schwartz, the thesis of which is that “eliminating consumer choices can greatly reduce anxiety for shoppers.”
Many thanks to Allen Downey and Q McCallum for feedback on drafts of this essay and to Cassie Kozyrkov for ongoing, thoughtful, and heated conversations about the topics covered.