DeepCheapFakes
What happens when deepfakes become cheap?
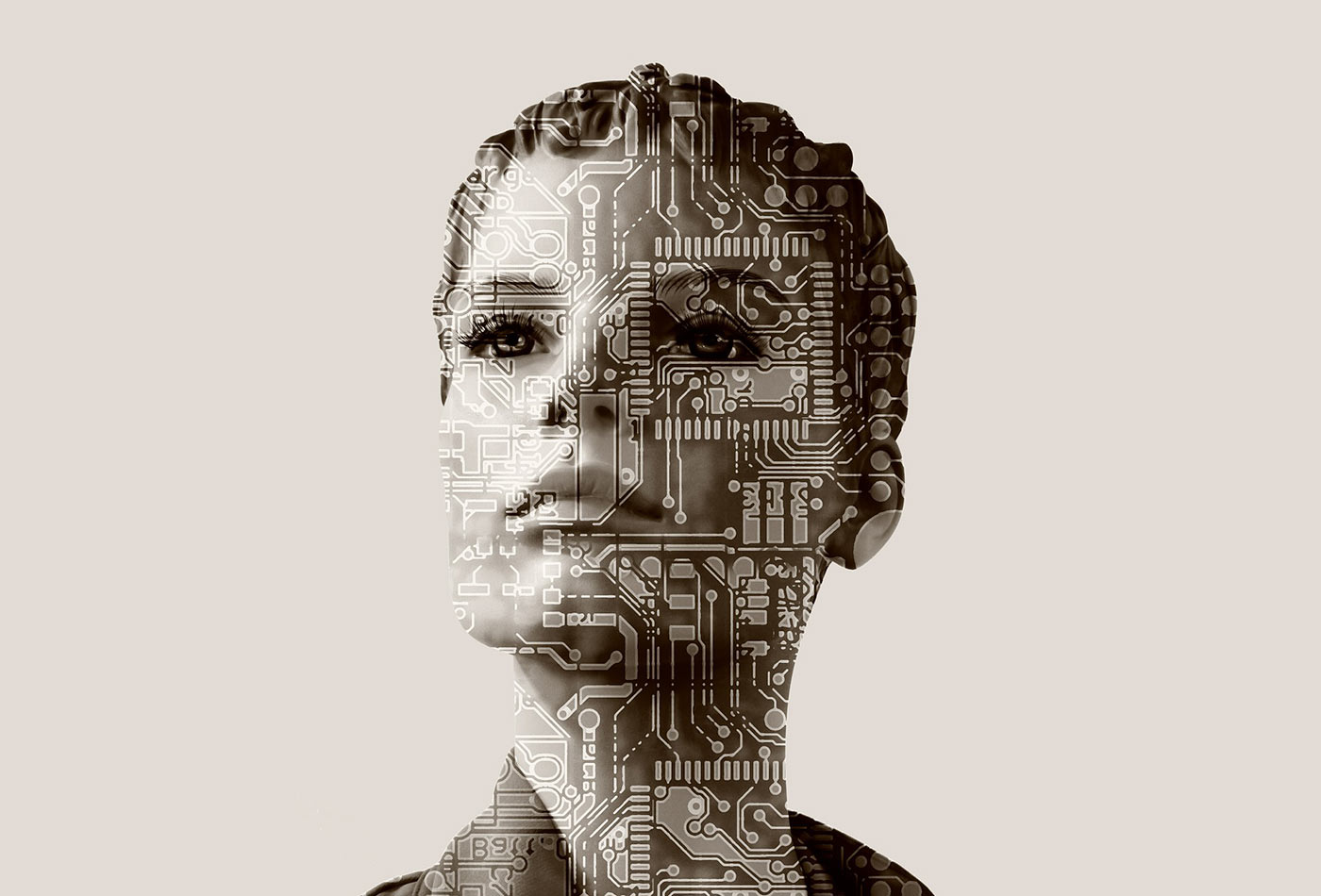
Back in 2019, Ben Lorica and I wrote about deepfakes. Ben and I argued (in agreement with The Grugq and others in the infosec community) that the real danger wasn’t “Deep Fakes.” The real danger is cheap fakes, fakes that can be produced quickly, easily, in bulk, and at virtually no cost. Tactically, it makes little sense to spend money and time on expensive AI when people can be fooled in bulk much more cheaply.
I don’t know if The Grugq has changed his thinking, but there was an obvious problem with that argument. What happens when deep fakes become cheap fakes? We’re seeing that: in the run up to the unionization vote at one of Amazon’s warehouses, there was a flood of fake tweets defending Amazon’s work practices. The Amazon tweets were probably a prank rather than misinformation seeded by Amazon; but they were still mass-produced.
Similarly, four years ago, during the FCC’s public comment period for the elimination of net neutrality rules, large ISPs funded a campaign that generated nearly 8.5 million fake comments, out of a total of 22 million comments. Another 7.7 million comments were generated by a teenager. It’s unlikely that the ISPs hired humans to write all those fakes. (In fact, they hired commercial “lead generators.”) At that scale, using humans to generate fake comments wouldn’t be “cheap”; the New York State Attorney General’s office reports that the campaign cost US$8.2 million. And I’m sure the 19-year-old generating fake comments didn’t write them personally, or have the budget to pay others.
Natural language generation technology has been around for a while. It’s seen fairly widespread commercial use since the mid-1990s, ranging from generating simple reports from data to generating sports stories from box scores. One company, AutomatedInsights, produces well over a billion pieces of content per year, and is used by the Associated Press to generate most of its corporate earnings stories. GPT and its successors raise the bar much higher. Although GPT-3’s first direct ancestors didn’t appear until 2018, it’s intriguing that Transformers, the technology on which GPT-3 is based, were introduced roughly a month after the comments started rolling in, and well before the comment period ended. It’s overreaching to guess that this technology was behind the massive attack on the public comment system–but it’s certainly indicative of a trend. And GPT-3 isn’t the only game in town; GPT-3 clones include products like Contentyze (which markets itself as an AI-enabled text editor) and EleutherAI’s GPT-Neo.
Generating fakes at scale isn’t just possible; it’s inexpensive. Much has been made of the cost of training GPT-3, estimated at US$12 million. If anything, this is a gross under-estimate that accounts for the electricity used, but not the cost of the hardware (or the human expertise). However, the economics of training a model are similar to the economics of building a new microprocessor: the first one off the production line costs a few billion dollars, the rest cost pennies. (Think about that when you buy your next laptop.) In GPT-3’s pricing plan, the heavy-duty Build tier costs US$400/month for 10 million “tokens.” Tokens are a measure of the output generated, in portions of a word. A good estimate is that a token is roughly 4 characters. A long-standing estimate for English text is that words average 5 characters, unless you’re faking an academic paper. So generating text costs about .005 cents ($0.00005) per word. Using the fake comments submitted to the FCC as a model, 8.5 million 20-word comments would cost $8,500 (or 0.1 cents/comment)–not much at all, and a bargain compared to $8.2 million. At the other end of the spectrum, you can get 10,000 tokens (enough for 8,000 words) for free. Whether for fun or for profit, generating deep fakes has become “cheap.”
Are we at the mercy of sophisticated fakery? In MIT Technology Review’s article about the Amazon fakes, Sam Gregory points out that the solution isn’t careful analysis of images or text for tells; it’s to look for the obvious. New Twitter accounts, “reporters” who have never published an article you can find on Google, and other easily researchable facts are simple giveaways. It’s much simpler to research a reporter’s credentials than to judge whether or not the shadows in an image are correct, or whether the linguistic patterns in a text are borrowed from a corpus of training data. And, as Technology Review says, that kind of verification is more likely to be “robust to advances in deepfake technology.” As someone involved in electronic counter-espionage once told me, “non-existent people don’t cast a digital shadow.”
However, it may be time to stop trusting digital shadows. Can automated fakery create a digital shadow? In the FCC case, many of the fake comments used the names of real people without their consent. The consent documentation was easily faked, too. GPT-3 makes many simple factual errors–but so do humans. And unless you can automate it, fact-checking fake content is much more expensive than generating fake content.
Deepfake technology will continue to get better and cheaper. Given that AI (and computing in general) is about scale, that may be the most important fact. Cheap fakes? If you only need one or two photoshopped images, it’s easy and inexpensive to create them by hand. You can even use gimp if you don’t want to buy a Photoshop subscription. Likewise, if you need a few dozen tweets or facebook posts to seed confusion, it’s simple to write them by hand. For a few hundred, you can contract them out to Mechanical Turk. But at some point, scale is going to win out. If you want hundreds of fake images, generating them with a neural network is going to be cheaper. If you want fake texts by the hundreds of thousands, at some point a language model like GPT-3 or one of its clones is going to be cheaper. And I wouldn’t be surprised if researchers are also getting better at creating “digital shadows” for faked personas.
Cheap fakes win, every time. But what happens when deepfakes become cheap fakes? What happens when the issue isn’t fakery by ones and twos, but fakery at scale? Fakery at Web scale is the problem we now face.