Overcoming barriers to AI adoption
The program for our Artificial Intelligence Conference in New York City will showcase tools, best practices, and use cases from companies leading the way in AI adoption.
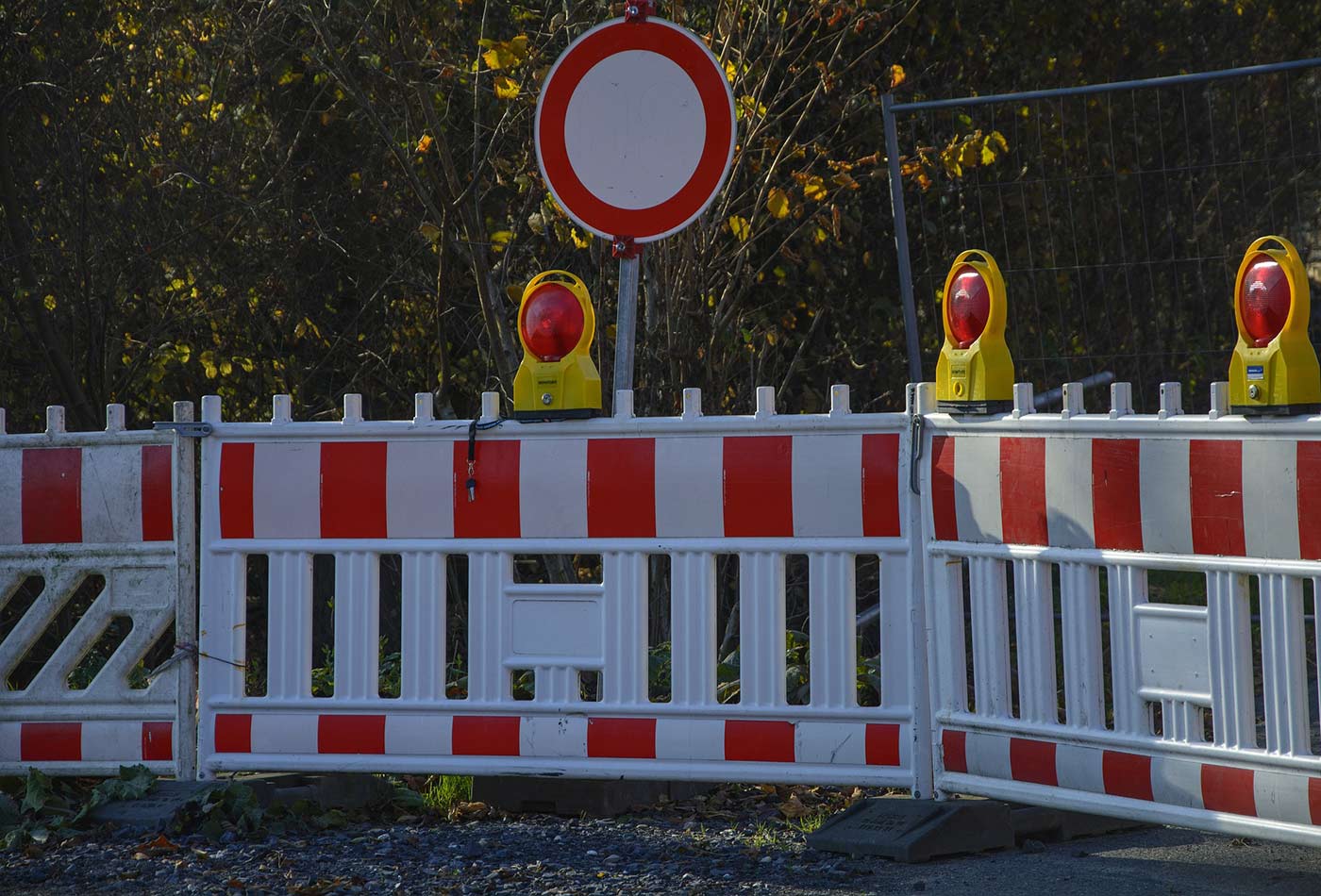
In early 2018, we conducted a survey to gauge the rate of adoption of deep learning. We found a majority of respondents were planning to use deep learning in future projects. When asked what held back adoption of deep learning, the same set of respondents cited “lack of skilled people,” “data-related challenges,” and “compute resources” as the main obstacles they faced.
We conducted another survey at the end of 2018, this time aimed at understanding adoption patterns for a broader set of AI technologies (not just deep learning). We found that companies that are just getting started using AI (what we termed the “evaluation stage”) cited company culture and difficulties identifying appropriate use cases as barriers to adoption. In contrast, those with more experience using AI technologies (what we termed a “mature practice”) cited “lack of data” and “lack of skilled people” as their main challenges.
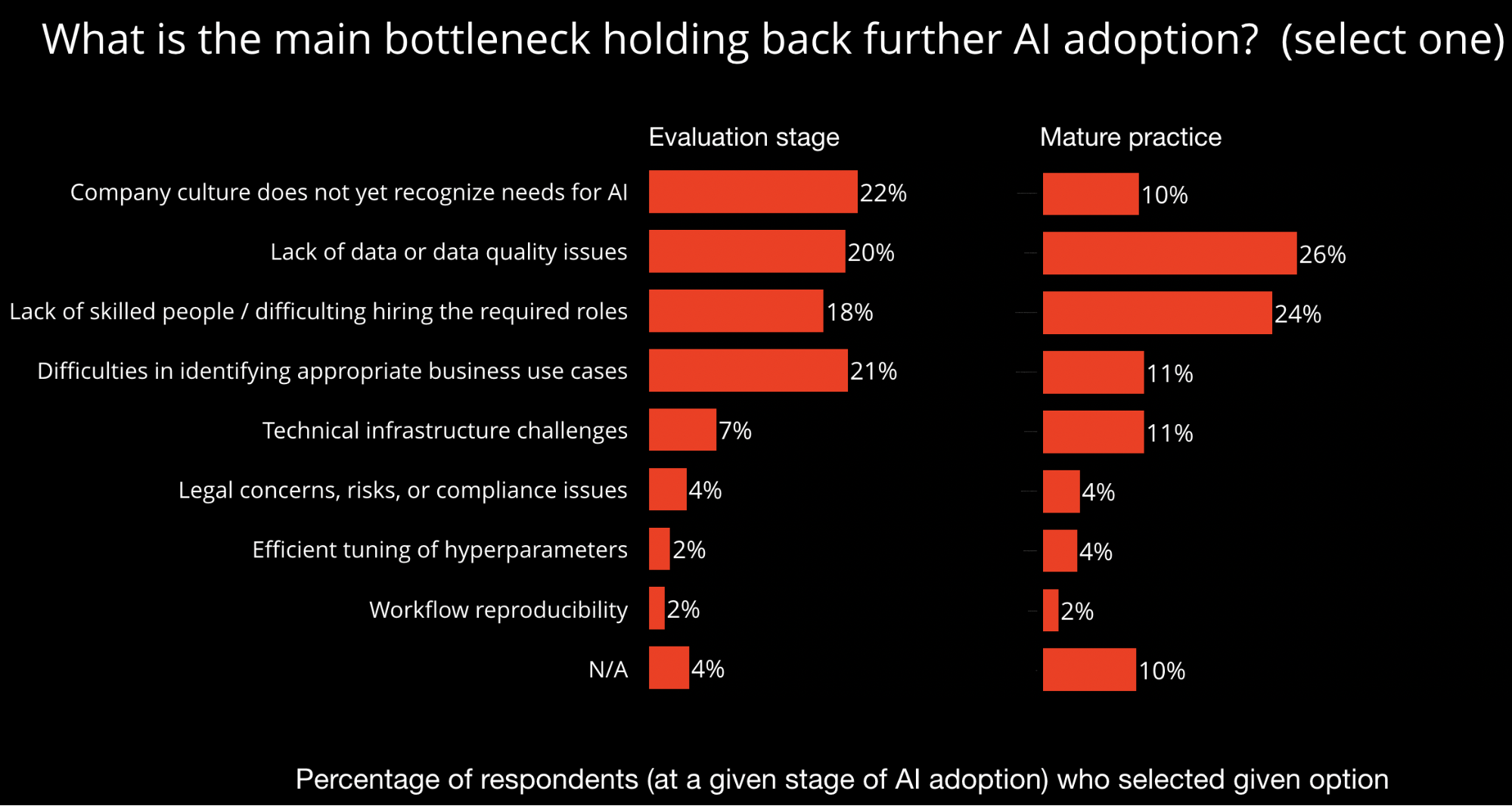
Much of the recent progress in research and tools is accessible to developers, and there are more instructional materials available as well. We are also beginning to see more case studies involving AI and automation technologies. Along with recent progress in models and algorithms, we’ll be showcasing tools, best practices, and use cases from leading companies at the Artificial Intelligence Conference in New York City, April 15-18, 2019.
Company culture and targeting the right use cases
As I noted in a recent post, there are many areas where current AI and automation technologies can already make an impact. We’ve assembled a series of training, tutorials, and briefings—the AI Business Summit—designed to help managers and executives develop playbooks for how to integrate AI technologies into existing workflows and products. Current AI and machine learning technologies require large amounts of data, so it makes sense for companies to investigate use cases in areas where they have existing data applications. This is precisely what we found in our upcoming survey, “Artificial Intelligence in the Enterprise”: for example, respondents from the financial services sector were already using AI in “customer service” or “finance and accounting.”
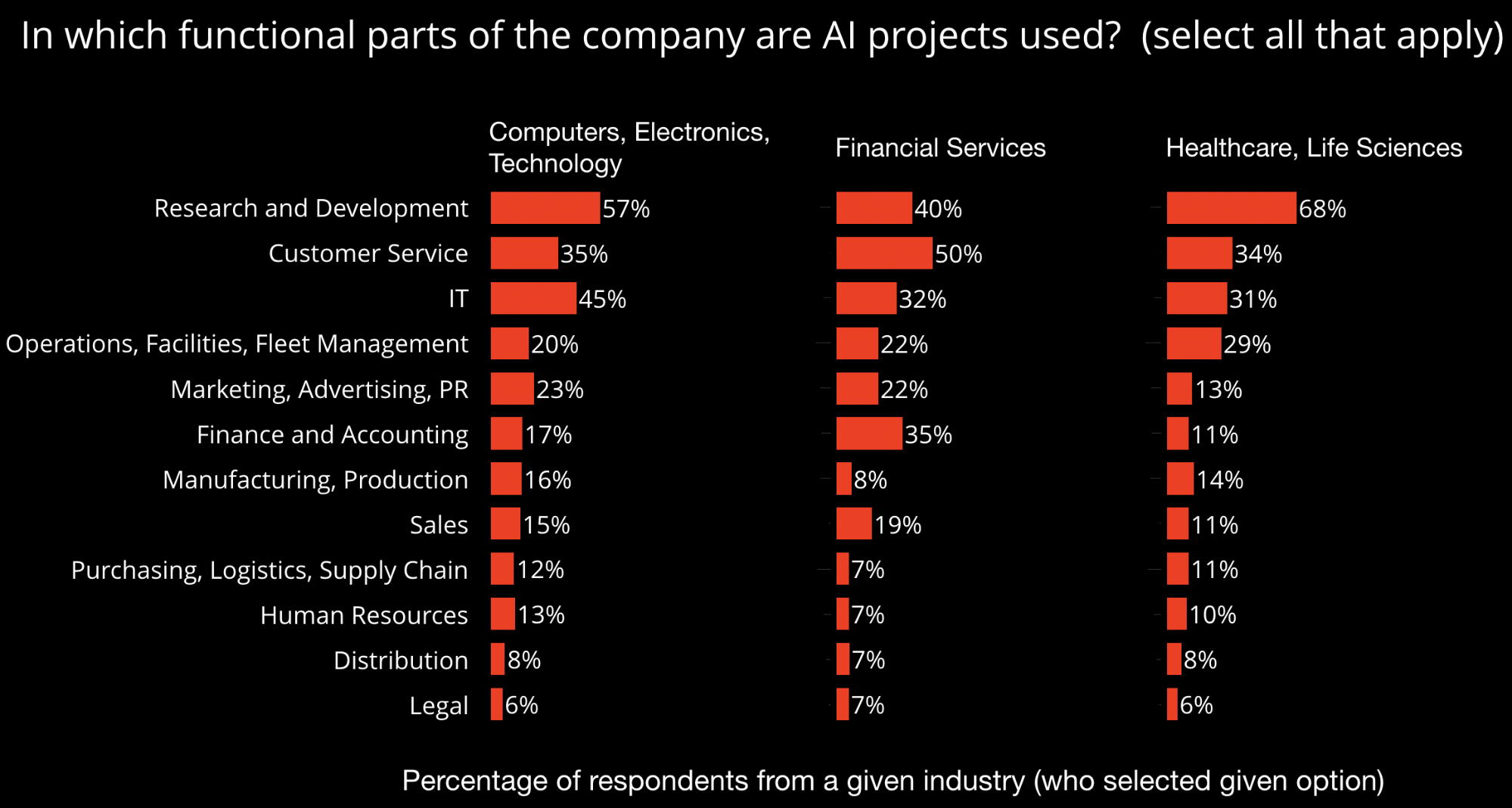
It’s also no surprise that even though deep learning is often associated with computer vision and speech technologies, we are beginning to see it used in areas where companies already have existing data sets and machine learning applications (specifically areas that involve text and time series). In fact, according to the survey results, structured data and text remain the main data types used for AI applications. One of the sessions at the conference will explore BERT, an exciting new language representation model that delivers state-of-the-art results in a wide range of natural language processing tasks.
Related training programs, tutorials, and sessions to explore at the Artificial Intelligence Conference in New York City include:
- AI Business Summit
- AI in the Enterprise
- Text, Language, and Speech
- Temporal data and time-series
- Computer Vision
There have been numerous articles written about artificial general intelligence, but the reality is that, at least for now, many of the AI systems that have captured press coverage have very specific and narrow capabilities. Entrepreneurs have taken notice. Many interesting AI startups are working on applications that are domain specific and target particular tasks and workflows. At the Artificial Intelligence Conference, we’ll have sessions and case studies from many industries, including:
The skills gap and lack of data
As I noted, respondents who work at organizations with more mature AI practices cited “data” and “lack of people” as the primary challenges they face as they attempt to adopt more AI technologies. When it comes to data, there are new tools designed to help companies overcome lack of data, including tools for generating synthetic data, simulation environments, and automation technologies designed to supercharge human labelers (Amazon Ground Truth is the most recent example).
In our upcoming survey, “Artificial Intelligence in the Enterprise,” we found companies at different stages of AI adoption are in need of talent across many skill sets. In particular, the need for skilled data and infrastructure engineers is the same across maturity levels:
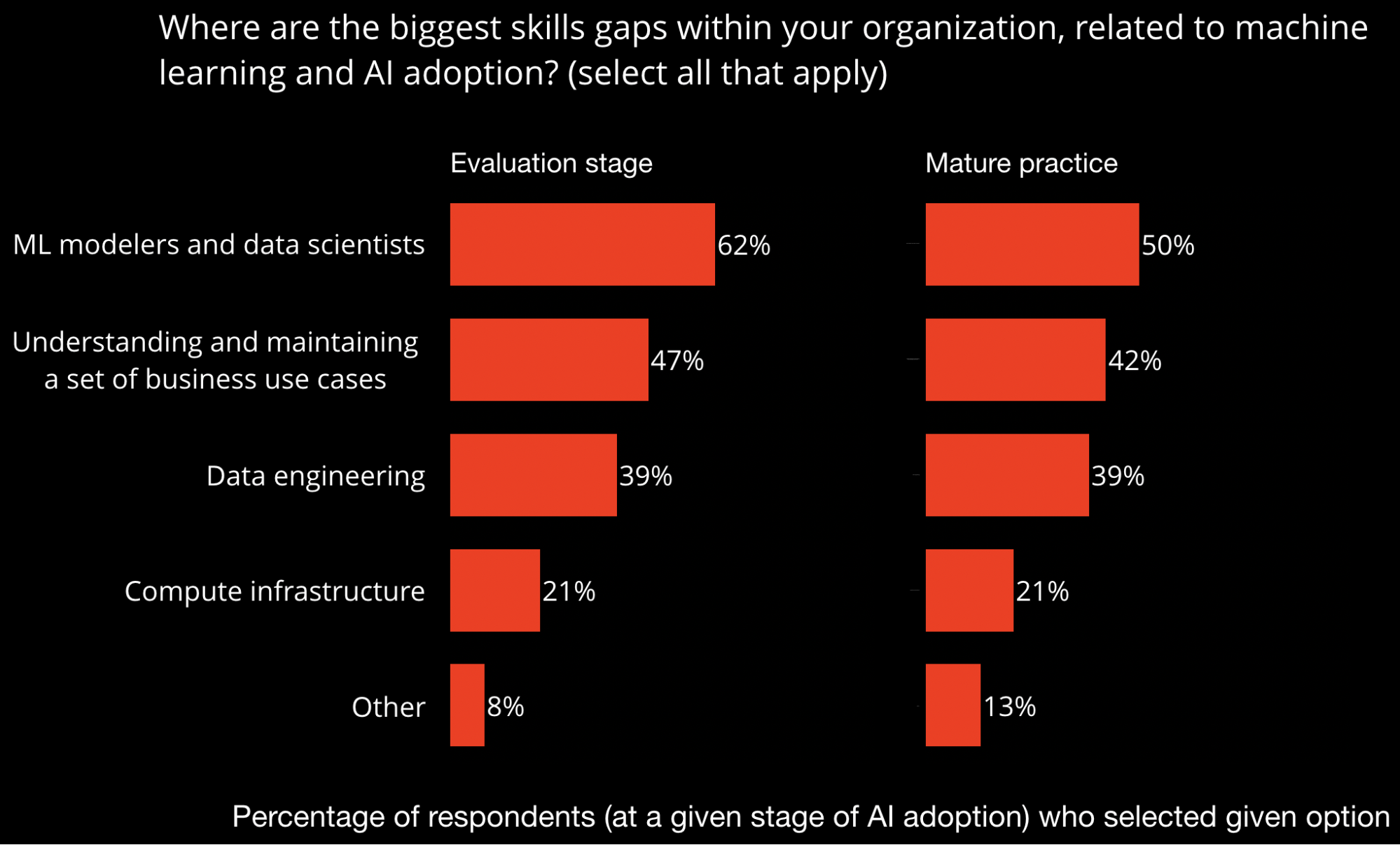
Improvements in tools have made AI more accessible for non-experts. Many machine learning libraries are open source, and many cutting-edge models (found in research papers) eventually get implemented in these libraries. In our previous survey, we found the top three deep learning tools to be TensorFlow (at the time, used by 61% of all respondents), Keras (25%), and PyTorch (20%). This year, we found a higher rate of usage for Keras (34%) and PyTorch (29%). We also are seeing improvements in tools for reinforcement learning: the most recent version of Ray now supports multi-agent reinforcement learning at large scale.
But AI requires a suite of technologies that go beyond machine learning libraries. To this end, companies are beginning to build or buy tools that can support and sustain their teams of data scientists and suites of AI applications. Leading companies will present some of their internal tools and platforms at the Artificial Intelligence Conference in April:
- Deep Learning and Machine Learning tools
- Platforms and infrastructure
- Models and Methods
- Reinforcement Learning
- Data and Data Networks
- “Open source tools for machine learning models and data sets versioning”
Responsible AI
In parallel with the progress in AI research and the progress in tools for building AI applications, there has been strong awareness around issues that go beyond simply optimizing business or quantitative metrics. This includes such topics as ethics, privacy and security, fairness, reliability and safety, and the economic impact of automation technologies. The community is beginning to come up with concrete strategies and best practices to address many of these concerns. For example, one of the sessions at the conference will provide a technical overview for a framework for Responsible AI used within Google. Additionally, there will be many other practical sessions at the Artificial Intelligence Conference from practitioners who are working at the forefront of designing AI applications that can overcome many of these important considerations: